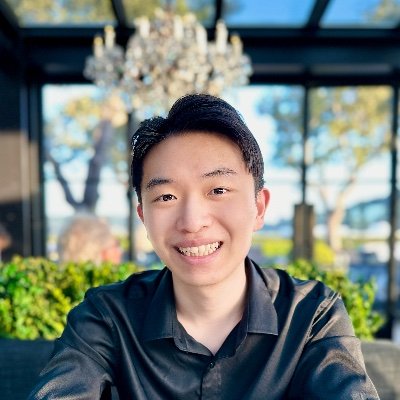
Jerry Wei
@JerryWeiAI
Followers
8K
Following
262
Media
48
Statuses
283
Aligning AIs at @AnthropicAI ⏰ Past: @GoogleDeepMind, @Stanford, @Google Brain
San Francisco, CA
Joined June 2015
Life update: After ~2 years at @Google Brain/DeepMind, I joined @AnthropicAI!. I'm deeply grateful to @quocleix and @yifenglou for taking a chance on me and offering me to join their team before I even finished my undergrad at Stanford. Because of their trust in my potential,.
33
41
1K
New @GoogleAI paper: How do language models do in-context learning? Large language models (GPT-3.5, PaLM) can follow in-context exemplars, even if the labels are flipped or semantically unrelated. This ability wasn’t present in small language models. 1/
14
208
949
New @GoogleAI paper! 📜. Language models repeat a user’s opinion, even when that opinion is wrong. This is more prevalent in instruction-tuned and larger models. Finetuning with simple synthetic-data ( reduces this behavior. 1/
12
137
614
New @GoogleDeepMind+@Stanford paper! 📜. How can we benchmark long-form factuality in language models?. We show that LLMs can generate a large dataset and are better annotators than humans, and we use this to rank Gemini, GPT, Claude, and PaLM-2 models.
9
78
372
Personal news: I've joined @GoogleDeepMind full-time as a researcher in @quocleix's and @yifenglou's team!. I've enjoyed the past eight months as a student researcher at Google Brain/DeepMind, and I'm excited to continue working on large language models and alignment! 😁.
15
2
337
My holiday side quest at @AnthropicAI: How well can Claude play Geoguessr? 🗺️. I had Claude look at 200K+ Street View images and guess the location. The results? Claude-3 models aren't that good, but Claude-3.5 models match or beat the average human!.
16
28
313
Fun fact: our paper was put on hold by arxiv for a while because arxiv detected that we used the phrase "time travel," which is a topic that arxiv frequently gets bad submissions for. When we Ctrl-F'd "time travel" in our paper, we had actually just cited a paper called "Time.
Google presents Best Practices and Lessons Learned on Synthetic Data for Language Models. Provides an overview of synthetic data research, discussing its applications, challenges, and future directions.
17
21
280
New @GoogleAI blog post summarizing the key experiments and results from our work!.
New @GoogleAI paper: How do language models do in-context learning? Large language models (GPT-3.5, PaLM) can follow in-context exemplars, even if the labels are flipped or semantically unrelated. This ability wasn’t present in small language models. 1/
1
36
199
A lot of people may underestimate Google, but we’ve now shown that we can ramp up quickly and achieve superb results. Bard now versus Bard one year ago is such a stark difference. Some may have even counted Google out of the fight when Bard was first released, but now they.
🔥Breaking News from Arena. Google's Bard has just made a stunning leap, surpassing GPT-4 to the SECOND SPOT on the leaderboard! Big congrats to @Google for the remarkable achievement!. The race is heating up like never before! Super excited to see what's next for Bard + Gemini
8
11
149
Interesting experimentation on how sycophantic OpenAI's base model are! An important counterfinding is that, like Anthropic models [1], PaLM base models also become more sycophantic as they get larger [2]. This difference between models may be explained by the fact that GPT-3
3
19
124
This is an awesome summary, thanks for reading our work elvis!.
Interesting findings on how LLMs do in-context learning. TL;DR: with scale, LLMs can override semantic priors when presented with enough flipped labels; these models can also perform well when replacing targets with semantically-unrelated targets.
0
10
115
Excited to share our latest research on making AI systems more robust against jailbreaks! 🚀. Our team at @AnthropicAI has developed Constitutional Classifiers, a new approach that significantly reduces vulnerability to jailbreaks while maintaining low refusal rates and.
New Anthropic research: Constitutional Classifiers to defend against universal jailbreaks. We’re releasing a paper along with a demo where we challenge you to jailbreak the system.
4
13
101
Excited to share that our work on symbol tuning (a method of improving in-context learning by emphasizing input-label mappings) was accepted to EMNLP! . See original tweet thread below👇.
New @GoogleAI+@Stanford paper!📜. Symbol tuning is a simple method that improves in-context learning by emphasizing input–label mappings. It improves robustness to prompts without instructions/relevant labels and boosts performance on algorithmic tasks.
2
12
89
Claude’s coding capabilities are underutilized - I think the new Sonnet’s coding abilities are incredibly strong to the point that I use Claude for a significant amount of my workflow. I would highly encourage everyone to at minimum try out the new Sonnet for coding!.
2
5
88
The new Sonnet achieves astounding performance at a sliver of the cost of competitors!. Very curious to see how it performs in the Chatbot Arena 🤓.
Introducing Claude 3.5 Sonnet—our most intelligent model yet. This is the first release in our 3.5 model family. Sonnet now outperforms competitor models on key evaluations, at twice the speed of Claude 3 Opus and one-fifth the cost. Try it for free:
1
2
74
New @GoogleAI research-blog post on our symbol tuning paper! 😁. Quick ~5 minute read summarizing the key methodology and findings. Find it here 👇.
Today on the blog, read all about symbol tuning, a method that fine-tunes models on tasks where natural language labels are replaced with arbitrary symbols. Learn how symbol tuning can improve in-context learning for benefits across various settings →
0
14
67
#NeurIPS should be held on a cruise ship next year. There's a lot of untapped potential here. 🤔.
4
2
64
This is one of the core surprising findings of our paper - previous efforts in using LLMs for evaluation primarily seek to achieve high correlation with human annotations. But we took a closer look at the data and noticed that human raters were not super reliable in fact.
LLM Agents Are Superhuman At Fact Checking. -LLM breaks down long texts into sets of individual facts.-Checks each fact w/ multi-step reasoning processes.-Using Google & determining whether fact is supported by the search results.-20x cheaper than humans.
0
5
66
Excited to share that I'll be at NeurIPS next week to discuss our work on evaluating long-form factuality! We'll have our poster session on Thursday (Dec 12) at 4:30 at Poster Session 4 East, and I'll also be floating around the conference/in the city. I'd be happy to meet other.
New @GoogleDeepMind+@Stanford paper! 📜. How can we benchmark long-form factuality in language models?. We show that LLMs can generate a large dataset and are better annotators than humans, and we use this to rank Gemini, GPT, Claude, and PaLM-2 models.
0
5
57
Arriving in Singapore for #EMNLP2023!. I’ll be discussing our work on symbol tuning - a simple finetuning method that improves a language model’s in-context learning abilities. Feel free to stop by during our poster sessions!. See original tweet thread below 👇.
New @GoogleAI+@Stanford paper!📜. Symbol tuning is a simple method that improves in-context learning by emphasizing input–label mappings. It improves robustness to prompts without instructions/relevant labels and boosts performance on algorithmic tasks.
1
2
56
Super excited for these improvements that the team has been working on! I'm particularly a fan of computer use, which is a natural next step towards allowing Claude to perform tasks like humans do 🤓.
Introducing an upgraded Claude 3.5 Sonnet, and a new model, Claude 3.5 Haiku. We’re also introducing a new capability in beta: computer use. Developers can now direct Claude to use computers the way people do—by looking at a screen, moving a cursor, clicking, and typing text.
1
2
54
Part of developing your own research brand is having a great website - a great website is more memorable to readers and helps them remember your work. Like many researchers, my website used to follow the typical Jon Barron layout (shoutout to @jon_barron for creating a simple.
1
1
49
There has been growing concerns about running out of high-quality training data for LLMs, and naturally many turn towards synthetic data to help remedy this issue. Indeed, synthetic data can be generated at large scales and is thus a valuable resource for training/evaluating.
Thanks Aran for sharing our work!. This is a survey paper I’ve been thinking about for a long time, as we have seen an increasing need for synthetic data. As we will probably run out of fresh tokens soon, the audience of this paper should be everyone who cares about AI progress.
1
8
49
Huge congrats to @YiTayML and the rest of the Reka team for this launch! Personally, I'm super impressed with how Reka-Core can match/beat GPT-4-Turbo and Claude-3-Opus on many benchmarks despite Reka being a much smaller team. Also "as for Belebele, we hit our credit threshold.
Our @RekaAILabs Tech Report / Paper is out! 🔥 . Tech reports with completely no information are kinda boring so we’re revealing some interesting information on how we train our series of Reka models including tokens, architecture, data & human evaluation workflows. 😃. We tried
2
1
33
Thanks for this insightful feedback!. Clarified these points in a revision:. 1. Replaced "superhuman" with "outperforms crowdsourced human annotators" to not imply beating expert humans.2. Added FAQ sec. discussing this distinction.3. Updated related work/SAFE with prior methods.
This is a cool method, but "superhuman" is an overclaim based on the data shown. There are better datasets than FActScore for evaluating this:.ExpertQA by @cmalaviya11 +al.Factcheck-GPT by Yuxia Wang +al (+ same methodology) 🧵.
0
1
31
Big thank you to my collaborators who worked with me on this paper! . @_jasonwei @YiTayML @dustinvtran @albertwebson @yifenglou @xinyun_chen_ @Hanxiao_6 @dhuangcn @denny_zhou @tengyuma . Thanks @sewon__min for providing super helpful feedback!. Paper: 9/.
5
1
31
Super cool work from @zorikgekhman and others at @GoogleAI!. Our team previously investigated fine-tuning LLMs to reduce sycophancy; one of our key findings was that you have to filter out prompts that the model does not know the answer to. The lesson we learned was that training.
Does Fine-Tuning LLMs on New Knowledge Encourage Hallucinations?. New preprint!📣. - LLMs struggle to integrate new factual knowledge through fine-tuning.- As the model eventually learns new knowledge, it becomes more prone to hallucinations😵💫. 📜 🧵1/12👇.
1
10
31
I’m super excited to see all of the interp work that could come out of this! Being able to steer frontier models opens up many directions of research that may otherwise have gone unexplored.🤓🤓.
Loved Golden Gate Claude? 🌉 . We're opening limited access to an experimental Steering API—allowing you to steer a subset of Claude's internal features. Sign up here: *This is a research preview only and not for production use.
0
0
30
Popular benchmarks like MMLU seem to be unable to truly measure a model’s capabilities due to overfitting and contamination concerns; there’s a growing need for new evals with unseen data that can allow us to quantify a model’s actual abilities. The LMSYS leaderboard is a step.
🚀 Introducing the SEAL Leaderboards! We rank LLMs using private datasets that can’t be gamed. Vetted experts handle the ratings, and we share our methods in detail openly! . Check out our leaderboards at . Which evals should we build next?
0
4
30
Arriving in Vienna for #ICLR2024! 🛬. I’ll be helping out at the GDM booth from 9-11AM on Tuesday and 2-3PM on Wednesday!. If you're interested in chatting about alignment/safety, hallucinations, factuality, or in-context learning, please stop by and say hi!😄.
1
1
25
Really excited to see this new eval on short-form factuality!. Factual language models should ideally only provide details that they are sure about. This means that in long-form settings, they should not try to make claims that might be incorrect, and in short-form settings, they.
Excited to open-source a new hallucinations eval called SimpleQA! For a while it felt like there was no great benchmark for factuality, and so we created an eval that was simple, reliable, and easy-to-use for researchers. Main features of SimpleQA:. 1. Very simple setup: there
0
3
24
@OrionJohnston @EricBuess @elder_plinius @janleike @alexalbert__ @skirano @AnthropicAI According to our server records, no one has jailbroken more than 3 levels so far.
Update: we had a bug in the UI that allowed people to progress through the levels without actually jailbreaking the model. This has now been fixed! Please refresh the page. According to our server records, no one has jailbroken more than 3 levels so far.
0
0
23
Welcome to the team!.
Personal news: I'm joining @AnthropicAI! 😄 Anthropic's approach to AI development resonates significantly with my own beliefs; looking forward to contributing to Anthropic's mission of developing powerful AI systems responsibly. Can't wait to work with their talented team,.
0
0
21
Clio is one of the coolest pieces of work I’ve seen in a while! There’s a lot of insights that can be learned from seeing how real users use Claude, and Clio does a great job of allowing us to capture some of these insights. Highly recommend taking a look 👇.
New Anthropic research: How are people using AI systems in the real world?. We present a new system, Clio, that automatically identifies trends in Claude usage across the world.
0
2
20
Really excited to see more great work from Yi!.
Personal / life update: I have returned to @GoogleDeepMind to work on AI & LLM research. It was an exciting 1.5 years at @RekaAILabs and I truly learned a lot from this pretty novel experience. I wrote a short note about my experiences and transition on my personal blog here.
2
0
20
Big thanks to all of my collaborators who helped me with this work! 🙏. @Hou_Le.@AndrewLampinen.@XiangningChen.@dhuangcn.@YiTayML.@xinyun_chen_.@yifenglou.@tengyuma.@denny_zhou.@quocleix. Paper: 9/.
1
0
19
Congrats to the Gemini team on this amazing launch! 🚀 🎉 . I’m personally super excited about Gemini’s multimodality capabilities, so I’m interested to see how people will end up using it!.
Introducing Gemini 1.0, our most capable and general AI model yet. Built natively to be multimodal, it’s the first step in our Gemini-era of models. Gemini is optimized in three sizes - Ultra, Pro, and Nano. Gemini Ultra’s performance exceeds current state-of-the-art results on
0
2
19
I’ll be presenting our work on symbol tuning today (December 8) at #EMNLP2023! Our poster session is at 2:00-3:30PM in the East Foyer - come by and say hi! 😄.
New @GoogleAI+@Stanford paper!📜. Symbol tuning is a simple method that improves in-context learning by emphasizing input–label mappings. It improves robustness to prompts without instructions/relevant labels and boosts performance on algorithmic tasks.
1
1
18
@kahnvex Thanks for the suggestion! Of course this is how I would implement it in practice, but I wanted people who don't write code to understand the joke that the fancy algorithm is just an if statement, which is less clear if there's no if statements.
0
0
15
Great work from Tu that tackles an important issue in LLMs! One of the hardest parts of evaluating factuality is the lack of ground truth answers that are available. FreshQA is a great step towards tackling this issue by providing ground truths for a diverse set of questions.
🚨 New @GoogleAI paper:. 🤖 LLMs are game-changers, but can they help us navigate a constantly changing world? 🤔. As of now, our work shows that LLMs, no matter their size, struggle when it comes to fast-changing knowledge & false premises. 📰: 👇
0
2
15
🧐 Our work is inspired from our previous findings ( and expands on prior work, combining the ideas from other studies:. 7/.
New @GoogleAI paper: How do language models do in-context learning? Large language models (GPT-3.5, PaLM) can follow in-context exemplars, even if the labels are flipped or semantically unrelated. This ability wasn’t present in small language models. 1/
1
1
15
This blog post is a great short read to learn the key findings from our paper "Larger language models do in-context learning differently"! 😁.
During in-context learning (ICL), models are prompted with a few examples of input-label pairs before performing a task on an unseen example. Read how larger language models do in-context learning differently & how this can change with their scale →
1
2
14