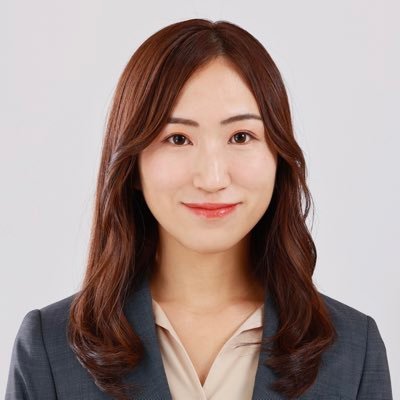
Akari Asai
@AkariAsai
Followers
15K
Following
9K
Media
118
Statuses
2K
Ph.D. student @uwcse & @uwnlp. NLP. IBM Ph.D. fellow (2022-2023). Meta student researcher (2023-). akariasai @ 🦋 I’m on academic job market this year!
Seattle, WA
Joined December 2017
🚨 I’m on the job market this year! 🚨.I’m completing my @uwcse Ph.D. (2025), where I identify and tackle key LLM limitations like hallucinations by developing new models—Retrieval-Augmented LMs—to build more reliable real-world AI systems. Learn more in the thread! 🧵
26
118
821
Don't miss our #ACL2023 tutorial on Retrieval-based LMs and Applications this Sunday! .with @sewon__min, @ZexuanZhong, @danqi_chen .We'll cover everything from architecture design and training to exploring applications and tackling open challenges! [1/2]
6
105
498
New work with Kazuma Hashimoto, @HannaHajishirzi, @RichardSocher, and @CaimingXiong at @SFResearch and @uwnlp! Our trainable graph-based retriever-reader framework for open-domain QA advances state of the art on HotpotQA, SQuAD Open, Natural Questions Open. 👇1/7
8
98
402
Retrieval-augmented LMs have made great progress& been adapted to real-world applications. Yet we still face major challenges. We @AkariAsai @sewon__min @ZexuanZhong @danqi_chen will be giving an ACL 2023 tutorial on retrieval-LMs! Join us to learn more about this exciting area.
2
39
295
Recently I gave a lecture about retrieval-augmented LMs like RAG, covering their advantages, an overview of diverse methods, and current limitations & opportunities, based on this position paper. .video: .Feedback is welcomed :).
𝗛𝗼𝘄 𝗰𝗮𝗻 𝘄𝗲 𝗯𝘂𝗶𝗹𝗱 𝗺𝗼𝗿𝗲 𝗿𝗲𝗹𝗶𝗮𝗯𝗹𝗲 𝗟𝗠-𝗯𝗮𝘀𝗲𝗱 𝘀𝘆𝘀𝘁𝗲𝗺𝘀? Our new position paper advocates for retrieval-augmented LMs (RALMs) as the next gen. of LMs, exploring the promises, limitations, and a roadmap for wider adoption. 🧵
6
52
283
New #acl2020nlp paper "Logic-Guided Data Augmentation and Regularization for Consistent Question Answerings"!.We show SOTA QA models produce inconsistent predictions and introduce logic-guided data augmentation & consistency-based regularization. 1/
2
47
263
Our paper got the ACL 2023 best video award (at EMNLP) 🥳🎉.The video by @alextmallen is available at .This 5 mins video summarizes the interesting findings on (1) when LLMs hallucinate (and scaling may not help) how retrieval-augmented LMs alleviate it.
Our work "When Not to Trust Language Models: Investigating Effectiveness of Parametric and Non-Parametric Memories" will appear in #ACL2023!!. This is my first NLP conference paper and I'm very happy I got to pursue this project with these amazing people at UW!.
5
25
265
This is a comprehensive list of the must-read papers on the recent progress of self supervised NLP models (or impressive capabilities of LLMs) and great summary slides! .I also love the role-playing paper-reading seminar fromat! 👩🏽🔬 (
For my first course at @jhuclsp, I am leading a class on recent developments in "self-supervised models." Here is the list of the papers and slides we cover: Would love to hear Twitter's suggestions for additional exciting developments to discuss!🤗.
3
55
255
Our #ICLR2020 camera-ready version, code, and blog are now available!.paper: code: blog: You can train, evaluate, and run an interactive demo on your machine. We also release the models for reproducibility.
New work with Kazuma Hashimoto, @HannaHajishirzi, @RichardSocher, and @CaimingXiong at @SFResearch and @uwnlp! Our trainable graph-based retriever-reader framework for open-domain QA advances state of the art on HotpotQA, SQuAD Open, Natural Questions Open. 👇1/7
2
48
236
📢 Thank you so much for attending our tutorial! 🙌 .🔗All the materials are available online .Our slide: The live Q&A on .If you registered for ACL, you can see the recorded Zoom video on Underline.
Don't miss our #ACL2023 tutorial on Retrieval-based LMs and Applications this Sunday! .with @sewon__min, @ZexuanZhong, @danqi_chen .We'll cover everything from architecture design and training to exploring applications and tackling open challenges! [1/2]
5
39
234
How GPT-4 triggers tools (eg web) seems to be similar to how Self-RAG works—fine-tuning an LM on augmented corpora with special tokens to call tools on demand during generation. We trained 7/13B LMs (open sourced) in this way.
@nbonamy It emits special words, e.g. <|BROWSE|> etc. When the code "above" the LLM detects these words it captures the output that follows, sends it off to a tool, comes back with the result and continues the generation. How does the LLM know to emit these special words? Finetuning.
1
24
230
Our work on scaling RAG with a 1.4T token corpus was accepted at @NeurIPSConf .This work led by @RulinShao has many interesting findings eg., .- RAG with massive corpus for better compute-optimal scaling.- thorough analysis on modeling / analysis choices at scale.Check it out!
We’ve known the importance of scaling pre-training data—what if we scale data used at “test time”? .Our new paper shows retrieval-augmented LMs can significantly benefit from our 1.4T token datastore on both up and downstream tasks & lead to better compute optimal scaling curve!.
2
29
220
Our BPR (ACL 2021, code is publicly available at Github) substantially reduces the index size (e.g., DPR consumes 65 GB while BPR only uses 2GB) without accuracy drop, and enables us to scale up to *billions of article* 📚.Great blog post on building a search engine using BPR!.
A great article on how to construct a 𝘣𝘪𝘭𝘭𝘪𝘰𝘯-𝘴𝘤𝘢𝘭𝘦 vector search engine using @vespaengine and our 𝗕𝗣𝗥 model! .
1
43
200
Our "Learning to Retrieve Reasoning Paths" paper has been accepted at #ICLR2020 !!! Joint work with amazing co-authors at @SFResearch and @uwnlp, Kazuma Hashimoto, @HannaHajishirzi, @RichardSocher, and @CaimingXiong :).
New work with Kazuma Hashimoto, @HannaHajishirzi, @RichardSocher, and @CaimingXiong at @SFResearch and @uwnlp! Our trainable graph-based retriever-reader framework for open-domain QA advances state of the art on HotpotQA, SQuAD Open, Natural Questions Open. 👇1/7
3
26
176
Had a great time at the NeurIPS instruction workshop and we are honored to get the Best paper Honorable Mention Award! . Check the details of the paper here: .
I will present Self-RAG at the instruction workshop (Room 220-222). - Poster sessioonn: 1 PM.- Oral talk: 4:50 PM .Come say hi! #NeurIPS2023.
5
10
167
Accepted at ACL2023 🇨🇦 as an oral paper! The updated camera ready version is available at ..LLMs hallucinate more in long tails, which scaling may not help while retrieval does. Adaptively combining retrieval w/ LLMs improves performance and efficiency.
Can we solely rely on LLMs’ memories (eg replace search w ChatGPT)? Probably not. Is retrieval a silver bullet? Probably not either. Our analysis shows how retrieval is complementary to LLMs’ parametric knowledge [1/N].📝 💻
0
23
151
Self-RAG is now available on @llama_index 🥳 .Also some update: Self-RAG is accepted as an Oral at #ICLR2024, and we are now working to update the draft & improve code bases now. Stay tuned!.
Self-RAG in @llama_index. We’re excited to feature Self-RAG, a special RAG technique where an LLM can do self-reflection for dynamic retrieval, critique, and generation (@AkariAsai et al.). It’s implemented in @llama_index as a custom query engine with
4
16
148
I am very excited to announce that new work with @HannaHajishirzi has been accepted to #acl2020nlp @aclmeeting. We investigate the inconsistencies of SOTA QA models' predictions and introduce FOL logic-guided data augmentation & consistency-based training regularization. [1/2].
4
4
141
Excited to attend #NeurIPS2024 in person! I’ll be presenting MassiveDS and CopyBench. Details below 🧵👇. Let’s catch up and chat about:.- LLMs & Retrieval-Augmented/Augmented LMs.- LLM Applications for science (e.g., OpenScholar) & others.- Ph.D./faculty apps. and more!
4
16
140
Super excited that our XOR QA paper has been accepted at #NAACL2021 🎉🎊 .We are updating the paper given the insightful feedback from reviewers. Stay tuned for the final version!.
Knowledge is not uniformly distributed across languages so an ideal open-retrieval QA model should search and retrieve multilingual resources. We propose a new task 𝐗𝐎𝐑 𝐐𝐀 with a new dataset 𝐗𝐎𝐑-𝐓𝐲𝐃𝐢 QA(40k newly annotated Qs in 7 languages) .�
5
13
136
I will be attending #NeurIPS2023 in person from Dec 11-15 to present . 1. RealtimeQA (Dataset track) . 2. Self-RAG (Workshop on instruction tuning ) . Come say hi! DM or email me if you wanna chat 💬.
0
24
132
If you are planning to apply for CS PhD program this year, you should definitely sign up for PAMS! .You’ll be matched with one CSE PhD student, have zoom meetings with them & get lots feedback on your SOP. I mentored some via this program, one of whom is now at UW CSE PhD 😎.
The PAMS Program application will go LIVE on September 10! Sign up for an alert to apply and be matched with a PhD mentor who will provide personalized feedback and guidance on your application journey.@uwcse @csenews
7
24
131
MITテクノロジーレビューの「Innovators Under 35」に選出いただきました!✨.Hallucinationsなど既存大規模言語モデルの持つ多くの課題に効果的に対応できるRAGなどのRetrieval-augmented LMsの発展と実応用に、引き続き全力で取り組んでいきます。.
🎉 Honored to be named one of MIT Technology Review’s Innovators Under 35 from Japan! 🚀 .Thrilled to advance retrieval-augmented and augmented LMs that go beyond their built-in limits, driving real-world reliability and impact.
5
7
128
This work has been accepted at the first @COLM_conf! .We introduce a novel taxonomy for diverse LLM hallucination & 1k human annotations for hallucinations of multiple LLM outputs, and train a new RAG for this challenging task (all open sourced). Work led by @mishrabhika 🥳.
🚨We all complain about LLM "hallucinations", but what are they? We study Automatic Fine-grained Hallucination Detection, with a novel taxonomy, a benchmark, and a 7B LM, surpassing ChatGPT in hallucination detection and editing .
0
12
128
One of my favorite posters today. It has so many great Japanese examples 😂 #EMNLP2022livetweet .In Japanese, "me" can be 俺 (masculine and casual), 僕 (less masculine and casual) and 私 (gender-neutral but more often used by women and official)
2
8
127
Amazing follow-up of Self-RAG by @ocolegro, which fine-tunes Self-RAG on top of Mistral-7b. Even stronger performance from the original LLama2-7b-based Self-RAG! .Also, a nice API to retrieve documents and generate using the Self-RAG model is provided! .
We encountered issues with accuracy in synthetic data generation. Fortunately, a great approach called self-rag was introduced by @AkariAsai et al. Introducing SciPhi-Self-RAG-Mistral-7B-32k. I fine-tuned the SciPhi model with this and more. The result is powerful + efficient.
5
18
116
Our RealTimeQA has been accepted at the NeurIPS (dataset)! Kudos to @jungokasai 🚀.LLM parametric memories get obsolete quickly. RealTimeQA is one of the earliest work demonstrating the effectiveness of retrieval augmentation + GPT3 to address this.
RealTime QA will be presented at #NeurIPS2023 (Datasets and Benchmarks Track) in New Orleans! See you there!
2
12
119
Thrilled to share that PANGEA, our open, state-of-the-art multilingual multimodal model (led by @xiangyue96 @yueqi_song), has been accepted to @iclr_conf #ICLR2025! 🎉 . Dive into the details in our paper: And try the demo here!
While Multimodal LLMs are advancing quickly, most training and eval data remains heavily English-centric, excluding many of the world's languages 🌍.We developed a new state-of-the-art Multilingual Multimodal LLM outperforming MOLMO and LLaMA 3.2!.[1/3] 🧵.
3
15
115
Heading to ICLR 2024! .I’ll present Self-RAG on Tue at 4:15pm Oral (Hall A8-9) and help presenting my amazing friends’ work, BTR on Friday .(details in threads 🧵) .Feel free to reach out to me via email or Whova if you wanna chat!.
Introducing Self-RAG, a new easy-to-train, customizable, and powerful framework for making an LM learn to retrieve, generate, and critique its own outputs and retrieved passages, by using model-predicted reflection tokens. 📜: 🌐:
4
11
113
We’ve known the importance of scaling pre-training data—what if we scale data used at “test time”? .Our new paper shows retrieval-augmented LMs can significantly benefit from our 1.4T token datastore on both up and downstream tasks & lead to better compute optimal scaling curve!.
🔥We release the first open-source 1.4T-token RAG datastore and present a scaling study for RAG on perplexity and downstream tasks! .We show LM+RAG scales better than LM alone, with better performance for the same training compute (pretraining+indexing).🧵
1
13
110
How can we inject entity knowledge into BERT? In our #EMNLP2020 work, we introduce a new entity-aware self-attention mechanism and a new pre-training objective, advancing SOTA on many tasks such as Entity Typing / QA / NER. Paper, code, and trained models are now available 👇.
Our @emnlp2020 paper “LUKE: Deep Contextualized Entity Representations with Entity-aware.Self-attention” is now available on arXiv! We present new pretrained contextualized representations that achieve SOTA on five datasets including SQuAD and CoNLL-2003.
0
14
103
SOTA models still struggle in information-seeking QA datasets such as NQ while they suppress humans on many MRC datasets. Our long paper .@aclmeeting quantifies modeling challenges and suggests several ways to improve future dataset collection. [1/6].
3
16
103
Want to reduce the passage index size for your open-domain QA system? We propose 𝗕𝗶𝗻𝗮𝗿𝘆 𝗣𝗮𝘀𝘀𝗮𝗴𝗲 𝗥𝗲𝘁𝗿𝗶𝗲𝘃𝗲𝗿 (𝗕𝗣𝗥) that learns to hash passage embeddings into compact binary codes (65GB --> 2GB!!🥰) without losing final open QA performance. #ACL2021NLP.
🚀Neural passage retrieval with substantially reduced memory size🚀. BPR presented in our #acl2021nlp paper drastically reduces the memory size of the SOTA retriever (DPR) without a loss of QA accuracy. Paper: Code/Model: 👇Threads
0
17
104
While Multimodal LLMs are advancing quickly, most training and eval data remains heavily English-centric, excluding many of the world's languages 🌍.We developed a new state-of-the-art Multilingual Multimodal LLM outperforming MOLMO and LLaMA 3.2!.[1/3] 🧵.
🌍 I’ve always had a dream of making AI accessible to everyone, regardless of location or language. However, current open MLLMs often respond in English, even to non-English queries!. 🚀 Introducing Pangea: A Fully Open Multilingual Multimodal LLM supporting 39 languages! 🌐✨
2
15
104
Super excited to host the first large-scale cross-lingual open-retrieval QA shared task in *14* languages (with prizes💰) at #NAACL2022 MIA! .We release all models&preprocessing codes, training data eg., neg/pos passages to train multilingual retrievers/readers, and results [1/2].
#NAACL2022 We released the test data for our cross-lingual open-domain QA shared task! .All baseline codes, models, training corpora, and intermediate & final results are now available at.Sign up today at .
2
27
99
New paper🚨 dense retriever often suffers from in domain (supervised) and out of domain (zero shot) tradeoff. While prior work tries more complex arch, scaling models or costly data generation, DRAGON trained with progressive multi-teacher training achieves SOTA with Bert-base!.
🎉 New Paper 🎉 Introducing DRAGON, our Dense Retriever trained with diverse AuGmentatiON. It is the first BERT-base-sized dense retriever (DR) to achieve state-of-the-art effectiveness on both supervised and zero-shot evaluations. Link: 1/7
1
14
97
I'll be attending #EMNLP2022 in-person from Dec 7-12. Say hi if you're around! .2 of my papers will be presented. Happy to chat about research (QA, multilingual NLP, retrieval-augmented LMs. etc) as well as PhD applications if you need help :) .🧵.
2
1
92
I will be attending the upcoming #ACL2023NLP #ACL2023 in person! I will be presenting a tutorial and a paper on retrieval-based LMs. If you have any interest in this area or simply want to chat, please feel free to reach out to me. [1/4].
4
3
92
I’ll attend COLM next week to present FAVA (Tuesday poster session 3 #1) with @mishrabhika.See details below! . If you’re interested in chatting about research, PhD applications …etc find me at COLM (I’ll be there all days) or send messages on Whova!
🚨We all complain about LLM "hallucinations", but what are they? We study Automatic Fine-grained Hallucination Detection, with a novel taxonomy, a benchmark, and a 7B LM, surpassing ChatGPT in hallucination detection and editing .
1
11
92
I'm a big fan of this work! Retrieval-based Multimodal Modeling seems to be quite an exciting area with many interesting open questions & applications!.
#ICML2023.Generative AI is hot🔥 We introduce *Retrieval-Augmented* Multimodal Modeling, a new technique that boosts text & image generation accuracy (prevents hallucination) while reducing training cost by 3x!. I’ll be presenting the poster on Tuesday 11am. Happy to chat!!
0
13
91
Just started my summer internship at @MetaAI in Seattle with @scottyih @riedelcastro 🥳 I’m SO exited to work with fantastic folks at Meta Seattle and London! .If you are in Seattle during summer or coming to Seattle to attend NAACL in person, let's hang out 🏔☀️.
1
0
84
Thanks, @jerryjliu0 for highlighting Self-RAG! .Retrieval+LLM pipelines are powerful yet several issues remain (eg irrelevant context, unnecessary/insufficient retrieval, unsupported generations). More work to address them can further improve retrieval-guided LLM systems.
Advanced RAG architectures need to implement dynamic retrieval 🔎. A *big* downside with naive top-k RAG is the fact that retrieval is static:.⚠️ Always retrieves a fixed number (k) regardless of query.⚠️ What if query requires more context (e.g. summarization) or less context
1
10
81
Excited to announce that CodeRAG-Bench has been accepted to @naaclmeeting #NAACL2025 (Findings)! 🥳 .We’ve developed a comprehensive benchmark to evaluate diverse retrievers and LMs for retrieval augmentation in code. Checkout our paper, benchmark here
CodeRAG has so much potential yet is underexplored. Our new benchmark and corpora enable rigorous evaluations of code retrieval and RAG! Key takeaway: Even SOTA models like GPT-4 or Claude 3 can significantly benefit from retrieving from open, large-scale corpora as a data store.
0
29
83
Did you know that more than 50% of questions were unanswerable in both Natural Questions and TyDiQA? . In a new preprint with @eunsolc (, We carefully analyze unanswerable questions in both datasets and attempt to identify the remaining headrooms. 👇 1/3.
1
10
80
We've updated the TART paper with some new results .💡 FLAN-T5-based TART-full improves previous TART (T03B).💡Negative sample ablations: our new instruction-unfollowing negatives help a lot in cross-task retrieval .🤗ckpts: .(code will be available soon!).
New paper 🚨 Can we train a single search system that satisfies our diverse information needs?.We present 𝕋𝔸ℝ𝕋 🥧 the first multi-task instruction-following retriever trained on 𝔹𝔼ℝℝ𝕀 🫐, a collections of 40 retrieval tasks with instructions! 1/N
1
3
76
Cool work benchmarking instruction-following retrieval systems! While our TART-dual ( performs well despite only having 110M params (best robustness and second best NDCG among models<7B), still these’s large room for improvements eg instruction sensitivity.
Can retrievers follow 📝instructions📝, including your intentions and preferences? 🧐.Introducing INSTRUCTIR, a benchmark for evaluating instruction following in information retrieval. [1/N]
1
9
76
CodeRAG has so much potential yet is underexplored. Our new benchmark and corpora enable rigorous evaluations of code retrieval and RAG! Key takeaway: Even SOTA models like GPT-4 or Claude 3 can significantly benefit from retrieving from open, large-scale corpora as a data store.
Introducing 🔥CodeRAG-Bench🔥 a benchmark for retrieval-augmented code generation!.🔗- Supports 8 codegen tasks and 5 retrieval sources.- Canonical document annotation for all coding problems.- Robust evaluation of retrieval and end-to-end execution
2
35
76
Our 𝕖𝕧𝕚𝕕𝕖𝕟𝕥𝕚𝕒𝕝𝕚𝕥𝕪-guided generator was accepted to appear at the NAACL 2022 main conference: #NAACL2022 @naaclmeeting 🥳 Huge thanks to my co-authors @HannaHajishirzi @nlpmattg !!.Stay tuned for the final camera-ready version.
A powerful retriever+pre-trained generator (eg. DPR+T5) often relies on spurious cues / generates hallucinations. Our 𝕖𝕧𝕚𝕕𝕖𝕟𝕥𝕚𝕒𝕝𝕚𝕥𝕪-guided generator learns to focus and generate on the right passages and shows large improvements in QA/fact verification/dialogue👇
0
11
76
I’m super excited to give an invited talk virtually at SIGIR workshop REML(retrieval-enhanced machine learning)🎙️on July 27th!.
@SIGIRConf @841io @bemikelive @danqi_chen @MohitIyyer In the afternoon, REML will host four invited talks by @AkariAsai (University of Washington), @lateinteraction (Stanford), @lauradietz99 (UNH), and Anirudh Goyal (University of Montreal). #SIGIR2023.
1
8
71
Introducing Wikipedia2Vec, an efficient and easy-to-use toolkit to learn and visualize the embeddings of words and entities from Wikipedia. Paper: Site:Demo: #emnlp2020 (demo) led by @ikuyayamada [1/3]
2
11
71
I’ll be at #NAACL2022 in-person! .- presenting at Wednesday QA oral session (2:15 PM-3:45 PM) . .- organizing an workshop on multilingual NLP this Friday .Hit me up! So excited to attend conference in person for the first time!
0
5
69
An up-to-date curated list of BERT-related papers (downstream task such as QA, probing, multilingual, model compression, domain-specific. etc) by @stomohide .
1
16
67
We're releasing a new multimodal QA dataset, MultiModalQA! MultiModalQA consists of about 30k multimodal questions, some of which require complex multihop reasoning across modalities (image, text, and table). Check the cool video by @AlonTalmor and come to our poster @iclr_conf!.
When was the painting with two touching fingers completed? We present MultiModalQA: Complex Question Answering over Text, Tables & Images! @iclr_conf @OriYoran,@amnoncatav,@LahavDan,@yizhongwyz,@AkariAsai,@gabriel_ilharco, @HannaHajishirzi,@JonathanBerant
0
9
68
We're excited to announce the NAACL 2025 Workshop on Knowledge-Augmented NLP! 🎉.We invite submissions on cutting-edge topics, including retrieval-augmented LMs, knowledge acquisition, LLM memorization, parametric knowledge, and more. 🗓 Deadline: February 15, 2025.
🎉Excited to announce the 4th Workshop on Knowledge-Augmented NLP at NAACL 2025 in New Mexico, USA! Submission deadline: Feb 15, 2025. Eager to reconnect with old friends and welcome new faces in the Knowledge NLP community!.#NAACL2025 #NLProc
0
3
67
Really cool work on multimodal retrieval! In TART, we propose a new formulation of retrieval with instructions and show the effectiveness of instruction tuning for generalization and alignment w/ users intents. Excited to see it works well in multimodal ✨.
We found that the instruction tuning is crucial for not only boosting models' performance but also enabling the model to generalize to unseen information retrieval tasks. The gain is dramatic in most of the tasks. This project was partly inspired by @AkariAsai's TART paper.
0
13
64
Top Trends of Graph Machine Learning in 2020 by Sergei Ivanov in @TDataScience Happened to find our ICLR paper on reasoning path retrieval is mentioned in this great article :) Yes, "Knowledge graphs become more popular"!.
0
7
59
Our first MIA (Workshop on Multilingual Information Access) #MIA_2022 will be held at NAACL! .We're excited to invite amazing speakers @aliceoh @gneubig @seb_ruder @aviaviavi__ Holger Schwenk, Kathleen McKeown (and more to come) as well as posters & a shared task. Stay tuned 🤩.
We're excited to announce the 🌎🌟 #MIA_2022 🌟🌍Workshop on Multilingual Information Access will be held at #NAACL2022 in Seattle!. Shared Task Competition + Speaker info coming soon!.
0
16
60
I’ll give a remote talk at UCL to present our recent work including our soon-to-be-arXived position paper! . I’ll discuss .1. promises and limitations of current retrieval-augmented LMs.2. how to advance them beyond simple RAG and a longer-term roadmap for further progress.
📢 #WITalk with Akari Asai (@AkariAsai) . 📕 Reliable, Adaptable, and Attributable LMs with Retrieval. 🗓️ 23 February, Friday, 2024 at 4 PM BST. ✍️Do not forget to sign up: #WITalk @ucl_wi_group @ai_ucl @uclcs.
2
3
60
Many recent papers evaluate prompted davinci-002/003 or turbo (ChatGPT) on widely-used datasets as zero-or few-shot models. While they are definitely impressive, I've been wondering if they have been trained on such datasets as a part of training. This is an important effort.
⚠️Did #ChatGPT cheat on your test? Probably yes. Many papers have evaluated ChatGPT on various benchmarks. However, it is important to consider that LLMs might have seen and memorized these datasets during pretraining. Read our latest blog post: 🧵1/5.
2
5
59
多くの研究論文でも使われているhugginface transformers (私も研究で使っています) の入門コースの日本語翻訳版が公開されています🎉.とてもわかりやすい内容なので自然言語処理をこれから始めたい方は是非チェックしてみてください.
Hugging Faceから日本へのお知らせです!. Hugging Faceコースの日本語翻訳を始めました。東北大学のStudent Ambassadorsの皆さんのお陰で第一章の翻訳が終了しました。.今後もコツコツと翻訳していきます。.是非コースを読んでHugging Face Tranformersについて学んで、使ってみてください!.
0
17
58
XOR QA @NAACLHLT 2021 camera-ready version is online! Website (&data): Code: We did some minor changes to the XOR-TyDi QA data and released a new version (v1.1). Please check&download the updated version 😉.
Knowledge is not uniformly distributed across languages so an ideal open-retrieval QA model should search and retrieve multilingual resources. We propose a new task 𝐗𝐎𝐑 𝐐𝐀 with a new dataset 𝐗𝐎𝐑-𝐓𝐲𝐃𝐢 QA(40k newly annotated Qs in 7 languages) .�
1
14
56
Honored to have my work & @techreviewjp Innovator Under 35 award featured by @UWCSE! .Huge thanks to my incredible collaborators & mentors for making this journey possible. Let’s keep pushing the boundaries of AI to tackle real-world challenges! 🌍👩💻 .
#AI hallucinations are a problem; @UW #UWAllen @uwnlp Ph.D. student @AkariAsai may have the answer. She was named a @techreviewjp Innovator Under 35 for her pioneering work to make #LLMs more transparent and useful—without making stuff up. #IU35 #AIforGood.
0
1
57
We will present this work tomorrow, Tuesday at 17:00-19:00 GMT / 10:00-12:00 PDT (Tuesday session 4)& 20:00-22:00 GMT / 13:00-15:00 PDT (Tuesday session 5)! .Please come say hi to our virtual poster session :) #ICLR2020.
Our #ICLR2020 camera-ready version, code, and blog are now available!.paper: code: blog: You can train, evaluate, and run an interactive demo on your machine. We also release the models for reproducibility.
2
6
55
Really important question! Multilingual datasets translated from English datasets are common, which often don’t reflect native speakers’ interests / linguistic phenomena. Still many languages often only have translated data only (our survey on this .
Translating benchmarks has become an in-vogue way to evaluate non-English capabilities of language models (see, for example, @MistralAI's latest model ) but people who are using a LLM in another language probably have different concerns and interests.
2
6
53
学部生での研究インターンでの学びと現在の博士課程留学 (MSR Internship アルムナイ Advent Calendar 2020) .@msraurjp さんに声をかけていただき、学部4年次のMicrosoft Research Asiaでのインターンと博士課程留学の最初の1+α年振り返りを含めたブログ記事を書きました。.
0
3
53
@rajammanabrolu I completely agree with this point, but I’m also concerned that it’s becoming increasingly difficult for students to get into Ph.D. programs w/o 3 strong letters. Many, esp. underrepresented students, lack access to research opportunities to secure them . I struggled with this.
4
3
50
I will present Self-RAG at the instruction workshop (Room 220-222). - Poster sessioonn: 1 PM.- Oral talk: 4:50 PM .Come say hi! #NeurIPS2023.
Introducing Self-RAG, a new easy-to-train, customizable, and powerful framework for making an LM learn to retrieve, generate, and critique its own outputs and retrieved passages, by using model-predicted reflection tokens. 📜: 🌐:
0
9
48
This Friday I will give a talk about our #NeurIPS2021 paper, “One Question Answering Model for Many Languages with Cross-lingual Dense Passage Retrieval”.Come join us :D.
🚨 Only two more days till our next SEA meetup w/ @AkariAsai (U. Washington) and Arnold Overwijk (Microsoft) about Dense Passage Retrieval!. Don't forget to sign up on our Meetup page to receive the Zoom link: See you on Friday! 😁.
2
6
43
As shown in the example, Self-RAG can perform diverse tasks / follow instructions as in standard instruction-tuned LMs + incorporate external knowledge when it enhances factuality & quality. Model checkpoints are available at: .
Self-RAG enables fine-grained on-demand control over the retrieval component, and it can be used together with instruction tuning to boost the factuality of a general-purpose model! . Please check this great work led by @AkariAsai !.
0
8
43
This is a great news but the example is a bit wrong (but interesting). するます is grammatically incorrect as when followed by an auxiliary verb ます (often added for politeness), する must be conjugated to し, so it should be します. I wonder if it’s common in MT to Japanese….
Last week, @MetaAI introduced NLLB-200: a massive translation model supporting 200 languages. Models are now available through the Hugging Face Hub, using 🤗Transformers' main branch. Models on the Hub: Learn about NLLB-200:
1
6
45
とても尊敬する友人の @00_ がアメリカ大学院出願に至る敬意を書いてくれています。海外大学院博士課程留学を目指している方もそうではない方も、是非読んでみてください。.「私生活を多少犠牲にし、同級生が遊んだり、就活や院試をしている間も研究や勉強をする決意をしてください。」.
留学を志した経緯を大学入学時から振り返りながら書きました。ご笑覧ください。. Posted to Hatena Blog.アメリカ博士課程留学 − 立志編 - yamaguchi.txt #はてなブログ.
0
3
43
明日の11時からAI王最終報告会で講演させていただきます。多言語での情報検索・質問応答を可能にするための大規模データセット・モデルの設計や今年NAACL2022ワークショップで行ったコンペティションの結果等についてお話しさせていたただく予定です。.
12/2(金)に開催されます,第3回 日本語質問応答コンペティション #AI王 では,浅井 明里 氏(University of Washington)から『より多くの言語での情報検索・質問応答を可能にするために』の招待講演を頂きます.貴重な機会ですので是非ご参加ください!.事前登録はこちら:
0
8
43
Inference-time scaling with Augmented LMs (eg tool usage, feedback from the environment) is even more powerful🔥Also surprisingly, the OpenHands agent picked up the tools, edited iteratively given feedback and visualized the results by itself with minimal instructions 👀.
Inference-time reasoning is definitely an interesting time topic, but tool usage and getting feedback from the environment seems essential as well. (and it can also make pretty plots like the one at the beginning of this thread :)).
1
8
42
𝐋𝐔𝐊𝐄, our deep contextualized word&entity representations, is now at huggingface transformers🤗 .You can reproduce the SOTA results on tasks like Open Entity easily at the colab notebooks..Paper&Video:
LUKE has just been added to the master branch of Huggingface Transformers!🎉🎉 It should be included in the next release of Transformers! Huge thanks to @NielsRogge and @huggingface folks!.
0
10
42
ACL2020: General Conference Statistics @aclmeeting ."To show how much our field has grown, ACL 2002 received 258 submissions total across all tracks." 😲
1
7
39