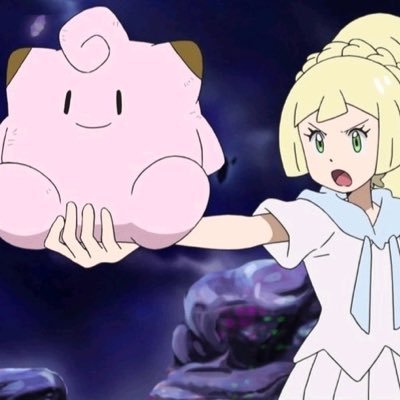
Zheng Yuan
@GanjinZero
Followers
870
Following
2K
Media
30
Statuses
556
NLP Researcher. The author of RRHF, RFT and MATH-Qwen. Focus on Medical & Formal & Informal Math & Alignment in LLMs. Prev @Alibaba_Qwen, Phd at @Tsinghua_Uni
Joined August 2013
@_lewtun I am one of the author in Qwen, I am sure no test set leakage in math related benchmark.
6
1
25
Scaling is all u need! Very similar to our observation in previous math sft scaling law and
Common 7B Language Models Already Possess Strong Math Capabilities. Mathematical capabilities were previously believed to emerge in common language models only at a very large scale or require extensive math-related pre-training. This paper shows that the LLaMA-2 7B model
1
2
17
I built the math related parts in Qwen. Trying to approach Minerva!.
Qwen-14B (Alibaba). The most powerful open-source model for it's size. And the longest trained: 3T tokens. Comes in 5 different versions:.Base, Chat, Code, Math and Vision. (And is even trained for tool usage!). Opinion: You should consider it as your new "go-to". ---.Paper:
0
0
14
Glad to be accepted by ICLR. Great job. @KemingLu612 @yiguyuan20.
📢 Check out our latest paper - 🏷️#INSTAG: INSTRUCTION TAGGING FOR ANALYZING SUPERVISED FINE-TUNING OF LARGE LANGUAGE MODELS! . 🔍 We propose 🏷️#INSTAG, an open-set fine-grained tagger for analyzing SFT dataset. 🔖 We obtain 6.6K tags to describe comprehensive user queries.
1
1
13
Yesterday I also saw a math reasoning paper work on iteratively generate new data for boosting. I believe using reward model ability inside the self model is a scalable way for improvement.
🚨New paper!🚨.Self-Rewarding LMs.- LM itself provides its own rewards on own generations via LLM-as-a-Judge during Iterative DPO.- Reward modeling ability improves during training rather than staying fixed. opens the door to superhuman feedback?.🧵(1/5)
1
1
7
Find this guy @KemingLu612 at nips instruction following workshop who do a lot on QWen alignment, instag(, and zooter(.
0
0
8
@yuntiandeng @KpprasaA @rolandalong @paul_smolensky @vishrav @pmphlt Our paper show the scaling law of augmented dataset amount vs performance on gsm8k.
1
1
6
Congratulate @kakakbibibi another work from us to investigate SFT data. We investigate data scaling curve for code, math, general abilities and how data composition influences each. We propose a dual-stage SFT to maintain math and code ability and have good general ability.
👏👏Excited to share our paper:. 🧐How Abilities in Large Language Models are Affected by Supervised Fine-tuning Data Composition. 📎 🤩From a scaling view, we focus on the data composition between mathematics, coding, and general abilities in SFT stage.
1
3
6
📈 We use tags to define complexity and diversity and we find that the complex and diverse SFT dataset leads to better performance! .🎯 We use #INSTAG as a data selector to choose 6K samples for SFT. Our fine-tuned TagLM-13B outperforms Vicuna-13B on MT-Bench.
1
1
5
LLMs seem just do imitation on cot instead of real thinking.
Some initial insights and I might be wrong. Obviously, LLMs are learning math in a different way than human beings. Humans tend to learn from textbooks and generalize better than LLMs. It seems to me that LLMs do need way more training data to actually understand math.
0
0
5
It includes gsm8k rft as additional training dataset 🤗.
Introducing: StarCoder2 and The Stack v2 ⭐️. StarCoder2 is trained with a 16k token context and repo-level information for 4T+ tokens. All built on The Stack v2 - the largest code dataset with 900B+ tokens. All code, data and models are fully open!.
0
0
5
VLLM will generate new tokens for LLM pretraining.
Finally, the top 1 vision feature is transcribing the text in image into text. -- you know that there are so many high-quality textbooks that are not yet digitalized, many of the are simply a scan. So you guess where the data for training the next generation model will be
0
1
5
Thank you tweeting our zooter! Zooter distills supervisions from reward models for query routing and inferences with little computational overhead.
Routing to the Expert: Efficient Reward-guided Ensemble of Large Language Models. paper page: The complementary potential of Large Language Models (LLM) assumes off-the-shelf LLMs have heterogeneous expertise in a wide range of domains and tasks so that
0
1
4
Handsome.
@jeremyphoward @rasbt @Tim_Dettmers @sourab_m Now @KemingLu612 is telling us how Qwen was built the winning A100 base model
0
1
4
Cite bunch of our paper ^_^.
Data Management For LLMs. Provides an overview of current research in data management within both the pretraining and supervised fine-tuning stages of LLMs. It covers different aspects of data management strategy design: data quantity, data quality, domain/task composition, and
0
0
4
Math is a good domain for researching how synthetic data can be used for LLM.
Would an AI that can win gold in the International Math Olympiad be capable of automating most jobs?. I say yes. @3blue1brown says no. Full episode out tomorrow:. "Math lends itself to synthetic data in the ways that a lot of other domains don't. You could have it produce a lot
0
2
4
So happy to find math reasoning SFT improves so fast.
The potential of SFT is still not fully unlocked!!!!! Without using tools, without continue pre-training on math corpus, without RLHF, ONLY SFT, we achieve SOTA across opensource LLMs (no use external tool) on the GSM8k (83.62) and MATH (28.26) datasets:
0
0
3
Now we have Arxiv version with a bibtex lol.
Qwen Technical Report. paper page: Large language models (LLMs) have revolutionized the field of artificial intelligence, enabling natural language processing tasks that were previously thought to be exclusive to humans. In this work, we introduce Qwen,
0
0
4
@StringChaos @WenhuChen Totally agree. I think the most effective tokens are SFT tokens and the less are pretrain tokens in scaling parameters. It is very interest to know how syn/rl tokens scaling parameters. Very interested to know why formal domains easier?.
2
0
3
@keirp1 Their math shepherd paper is a very good start point of building prm. I very like that paper.
0
0
3
@doomslide @iammaestro04 @teortaxesTex @QuintinPope5 Translation is so hard but worth taking effort. LEAN and natural language are reasoning in different granularity. So many obvious things need to be proved by LEAN tactics.
1
0
3
With scaling law predictions.
@generatorman_ai Better data engineering.Scrapping textbooks.Codex as a distinct target.More serious attitude to getting to a commercially viable product.Not even a moment's hesitation about it being more than experiment.
0
0
3
RRHF accepted by @NeurIPSConf.
We just released the weights of our RRHF-trained Wombat-7B and Wombat-7B-GPT4 on Github and Huggingface.
0
0
2
Want see someone successful using PPO (with a reward model) to improve math reasoning.
I got a crazy theory about RLHF that I would like to debate about. No nice way to put it:.I am not sure RLHF was used for training GPT-3.5 and GPT-4. Please change my mind. Arguments:. -----.Supervised learning can go much farther than anyone thought it could. RLHF was never.
1
0
1
@KemingLu612 @yiguyuan20 Another problem is long data means long responses means better alignment performance.
0
0
2
@rosstaylor90 If people are comparing aligned models, I think it is fair to use expert iteration.
1
0
2
@sytelus @Francis_YAO_ @_akhaliq 1. r_ij means j selected paths after rejecting with k=100, usually it has like 5 paths. 2. Exactly.
0
0
2
@polynoamial @OpenAI My plan for improving MATH is iteratively improve policy model and reward model by RL.
0
0
1
This problem also appears in the test set of MATH benchmark. It’s time for LLMs to solve this problem now.
When I was in middle school I qualified for Nationals at MathCounts. and I remember distinctly watching @ScottWu46 (CEO of Cognition), absolutely destroy in the Countdown round. That was when I realized I was very very good at math, but I was not Scott
0
0
1