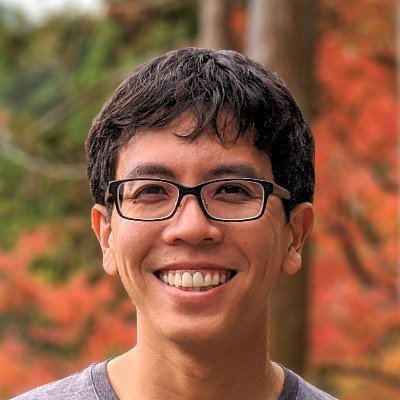
Pang Wei Koh
@PangWeiKoh
Followers
4K
Following
1K
Media
13
Statuses
309
Assistant professor at @uwcse and visiting research scientist at @allen_ai. Formerly @StanfordAILab @GoogleAI @Coursera. 🇸🇬
Joined June 2020
Honored to be named an #AI2050 Early Career Fellow by @schmidtsciences. I'm grateful to get to work on this research with all of my amazing students and wonderful colleagues at @uwcse and @allen_ai!.
We're thrilled to welcome the 2024 cohort of AI2050 Senior and Early Career Fellows –– 25 visionary researchers tackling AI's toughest challenges to ensure it serves humanity for the better. Learn more about this year’s cohort of fellows:.
17
11
149
We’re presenting WILDS, a benchmark of in-the-wild distribution shifts, as a long talk at ICML!. Check out @shiorisagawa's talk + our poster session from 9-11pm Pacific on Thurs. Talk: Poster: Web: 🧵(1/n)
4
20
98
@HannaHajishirzi and I are looking for postdocs/students to work on AI for science, including foundation models for scientific literature (as in + scientific data (genomics, images, molecules, . ). Let us know if interested and please help RT! 🧪.
2
18
96
If you're working on distribution shifts in ML -- and especially if you're working on ML applications with natural shifts -- please consider submitting your work to the NeurIPS Workshop on Distribution Shifts. Details below!.
We’ll be organizing a NeurIPS Workshop on Distribution Shifts! We’ll focus on bringing together applications and methods to facilitate discussion on real-world distribution shifts. Website: Submission deadline: Oct 8.Workshop date: Dec 13
0
13
53
Very excited to release OpenScholar, led by @akariasai! I've been using it to answer my own research questions, and we hope it'll be helpful for you too. We've open-sourced all models/code/data. Check out our demo below!.
1/ Introducing ᴏᴘᴇɴꜱᴄʜᴏʟᴀʀ: a retrieval-augmented LM to help scientists synthesize knowledge 📚 @uwnlp @allen_ai .With open models & 45M-paper datastores, it outperforms proprietary systems & match human experts. Try out our demo!.We also introduce ꜱᴄʜᴏʟᴀʀQᴀʙᴇɴᴄʜ,
0
5
45
2.5 weeks left to submit to the NeurIPS Workshop on Distribution Shifts! We especially welcome submissions on shifts in practice + areas and approaches not frequently represented at NeurIPS. Website: Apply to be a mentor/mentee by: Sep 22.Submit by: Oct 8.
We’ll be organizing a NeurIPS Workshop on Distribution Shifts! We’ll focus on bringing together applications and methods to facilitate discussion on real-world distribution shifts. Website: Submission deadline: Oct 8.Workshop date: Dec 13
0
10
43
Our WILDS benchmark is out! It was a real privilege to work with this amazing team + the many others who helped us along the way. We hope you'll find it useful. Please let us know if you have questions/feedback/suggestions -- or if you'd like to contribute a dataset!.
We're excited to announce WILDS, a benchmark of in-the-wild distribution shifts with 7 datasets across diverse data modalities and real-world applications. Website: Paper: Github: Thread below. (1/12)
0
6
42
@2plus2make5 Aw, thanks Emma. I didn't do much: @fereshte_khani brought this up at one of @percyliang's quarterly D&I lab meetings, and I just relayed it onwards. It was a tiny step and there's obvs lots left to do, but I'm glad to see people speaking up for these students now.
0
0
39
Check out JPEG-LM, a fun idea led by @XiaochuangHan -- we generate images simply by training an LM on raw JPEG bytes and show that it outperforms much more complicated VQ models, especially on rare inputs.
👽Have you ever accidentally opened a .jpeg file with a text editor (or a hex editor)?. Your language model can learn from these seemingly gibberish bytes and generate images with them!. Introducing *JPEG-LM* - an image generator that uses exactly the same architecture as LLMs
2
4
41
Most evals assume all the relevant info is given up front. What if models first have to ask questions to elicit this info? @StellaLisy showed SOTA models really struggle here; much more work is needed for reliable interactive models. Check out our MediQ benchmark at NeurIPS!.
31% of US adults use generative AI for healthcare🤯But most AI systems answer questions assertively—even when they don’t have the necessary context. Introducing #MediQ a framework that enables LLMs to recognize uncertainty🤔and ask the right questions❓when info is missing: 🧵
0
8
40
Instead of scaling pretraining data, can we scale the amount of data available at inference instead? Scaling RAG datastores to 1.4T tokens (on an academic budget) gives us better training-compute-optimal curves for LM & downstream performance. Check out @RulinShao's work below!.
🔥We release the first open-source 1.4T-token RAG datastore and present a scaling study for RAG on perplexity and downstream tasks! .We show LM+RAG scales better than LM alone, with better performance for the same training compute (pretraining+indexing).🧵
0
1
34
Our COVID paper is out in @nature today!! Huge thanks to @JacobSteinhardt @tatsu_hashimoto @shiorisagawa @percyliang for all of your help -- grateful to be in such a supportive lab, and for the chance to work with the absolutely amazing team below to contribute our little bit.
Check out our new paper in @nature, “Mobility network models of COVID-19 explain inequities and inform reopening”! @2plus2make5 @PangWeiKoh @jalinegerardin @beth_redbird @davidgrusky @jure 1/9
2
4
31
Reminder: Submissions to the NeurIPS DistShift workshop are due next Monday (Oct 3), AoE!.
We're organizing the second Workshop on Distribution Shifts (DistShift) at #NeurIPS2022, which will bring together researchers and practitioners. Submission deadline: Oct 3 (AoE).Workshop date: Dec 3.Website:
1
8
32
OLMoE, our fully open mixture-of-experts LLM led by @Muennighoff, is out! Check out the paper for details on our design decisions: expert granularity, routing, upcycling, load balancing, etc.
Releasing OLMoE - the first good Mixture-of-Experts LLM that's 100% open-source.- 1B active, 7B total params for 5T tokens.- Best small LLM & matches more costly ones like Gemma, Llama.- Open Model/Data/Code/Logs + lots of analysis & experiments. 📜🧵1/9
3
0
29
WILDS v1.1 is out! Training is much faster now, so it's easier to develop across multiple datasets; and check out the new Py150 code completion dataset, which tests the robustness of language models. Thank you to everyone who beta-tested this version and gave us feedback!.
We’ve released v1.1 of WILDS, our benchmark of in-the-wild distribution shifts! This adds the Py150 dataset for code completion + updates to existing datasets to make them faster and easier to use. Website: Paper: Thread 👇. (1/8)
1
4
28
Wow, just saw this video review on our concept bottleneck models paper. It's more detailed than any talk I've given on it. Thank you @AndreiMargeloiu for the great exposition. (@thao_nguyen26, who is joint first author with Yew Siang Tang, is applying to PhD programs this year!).
New Paper Review📹! Concept Bottleneck Models achieve competitive accuracy with standard end-to-end models while enabling interpretation in terms of high-level clinical concepts. @PangWeiKoh @2plus2make5 @_beenkim @percyliang .
1
4
27
So excited (and relieved) that WILDS v1.2 is out! We added 2 datasets: GlobalWheat, on wheat head detection, and RxRx1, on genetic perturbation classification. We also substantially updated the paper, esp. Sec 5 on measuring in-dist vs. out-of-dist gaps. Please check it out!.
Just in time for ICML, we’re announcing WILDS v1.2! We've updated our paper and added two new datasets with real-world distribution shifts. Website: Paper: ICML: Blog🆕: . 🧵(1/9).
0
3
28
Tune in tomorrow to catch @shiorisagawa discussing her work on distribution shifts, including DRO methods and the WILDS benchmark, at @trustworthy_ml's Rising Star series🌟.
1/ It’s Rising Star Spotlights Seminar ⭐️ time again! For this week’s TrustML seminar, we're delighted to host @shiorisagawa (Stanford) & @p_vihari (IITB) on Thurs Aug 19th 12pm ET 🎉🎉🥳. Register here: . See this thread for the speaker & talk details👇
0
4
27
Copyright discussions around LMs have focused on literal copying of training data. With CopyBench, we show that non-literal copying is even more pervasive but overlooked by current mitigation methods. Check out Tong's tweet for the details!.
📢Check out CopyBench, the first benchmark to evaluate non-literal copying in language model generation! .❗️Non-literal copying can occur even in models as small as 7B and is overlooked by current copyright risk mitigation methods. 🔗[1/N]
0
7
28
@2plus2make5 @leah_pierson As a sophomore, @AndrewYNg encouraged me to shadow clinicians, which gave me the chance to listen to them debate cases at a tumor board; and years later, the case-based reasoning they used was the motivation for @percyliang and I to write our paper on influence functions!.
2
1
24
Check out Shiori's invited talk tmr on WILDS v2 at the #ICML2022 PODS workshop! WILDS v2 adds unlabeled data from real-world distribution shifts -- come by to hear her discuss the benchmark and our takeaways from evaluating domain adaptation and self-supervised algorithms on it!.
I'm excited to speak at the Principles of Distribution Shift workshop at #ICML2022 tomorrow at 9:50am in Ballroom 3! I'll be talking about extending the WILDS benchmark with unlabeled data. Please join us!. The talk will also be streamed at
0
1
22
Last call for submissions to the NeurIPS Workshop on Distribution Shifts, due this Friday, Oct 8, at 11:59pm AoE! . Methods, theory, and empirical studies of distribution shifts are all welcome.
We’ll be organizing a NeurIPS Workshop on Distribution Shifts! We’ll focus on bringing together applications and methods to facilitate discussion on real-world distribution shifts. Website: Submission deadline: Oct 8.Workshop date: Dec 13
0
6
20
On the front page of the NYT today: @iarynam from @nytopinion wrote a really cool interactive article exploring predictions from our COVID model. It also visualizes the impact of different reopening policies on low- vs. high-income neighborhoods. Check out her work below!.
Super interesting opinion piece by @iarynam which dives.into our COVID model’s findings around capacity caps and socioeconomic disparities in the first wave of the pandemic. Love these gorgeous graphics; I’m definitely taking notes for my next data viz 😍.
0
2
19
@serinachang5, @2plus2make5, and I will present our COVID model (joint work with @jalinegerardin, @beth_redbird, @davidgrusky, @jure) at #ML4H in #NeurIPS2020. How is ML related to our work, and what kinds of ML research might improve it? . (Paper: . 1/.
1
3
15
Cool work by @rose_e_wang showing in an RCT that math tutors using Tutor CoPilot are significantly more likely to help students master their topic! Really nice to see these sorts of rigorous human-AI studies now.
AI has the potential to transform real-world domains. But can AI actually improve outcomes in live interactions?. We conducted the first large-scale intervention of a Human-AI Approach that has statistically significant positive learning gains w/ 900 tutors & 1,800 K12 students.
0
2
13
In addition, we'll have 4 spotlight talks on: .- A diabetic retinopathy benchmark (@neilbband, @timrudner et al.).- Distribution shifts on graphs (Ding, @KezhiKong, Chen et al.).- Contextual bandits (Qin and @DanielRuss0).- Importance weighting (@KAlexanderWang et al.).
1
2
8
@2plus2make5 @leah_pierson @AndrewYNg @percyliang Although given that our paper was mainly about dogs and fish, some of the real-world impact might have been lost along the way. .
0
0
8
@serinachang5 & collaborators at UVA turned our academic COVID model into a decision support tool that could actually be used by policymakers. This was a ton of important but not-always-glamorous work and it was really impressive to see how creative and careful she is. Congrats!!.
So excited to announce that we won the KDD 2021 Best Paper Award in the Applied Data Science track for our paper, "Supporting COVID-19 policy response with large-scale mobility-based modeling”!
1
0
8
Sara's work is an extremely impressive combination of advances in ML + real progress on challenges in conservation and sustainability. She's on the job market this year!.
🌍I'm on the job market🌎.I develop computer vision methods to enable global-scale biodiversity monitoring and conservation, tackling real-world challenges including strong spatiotemporal correlations, imperfect data quality, fine-grained categories, and long-tailed distributions.
0
1
7
@aliceoh @sunipa17 @kaiwei_chang @TristanNaumann @IAugenstein @computermacgyve @CamachoCollados @seo_minjoon @NoSyu @mohitban47 @VioletNPeng It was a real pleasure to attend; thank you Alice and all the amazing students for organizing this!.
0
0
7
Yay! So well deserved!.
Honored to have been named to MIT @techreview's 35 Innovators Under 35. Very grateful to the mentors and friends who collaborated on the work this award recognizes!!!.
0
0
5
This was joint work with @shiorisagawa* @tonyh_lee* IrenaGao*, and @sangmichaelxie @kendrick_shen @ananyaku @weihua916 @michiyasunaga HenrikMarklund @sarameghanbeery @EtienneDavid @IanStavness @guowei_net @jure @kate_saenko_ @tatsu_hashimoto @svlevine @chelseabfinn @percyliang.
2
0
6
HenrikMarklund @sangmichaelxie MarvinZhang @_bakshay @weihua916 @michiyasunaga @rlanasphillips IrenaGao TonyLee @EtienneDavid @IanStavness @guowei_net @bertonearnshaw @ImranSHaque @sarameghanbeery @jure @anshulkundaje @2plus2make5 @svlevine @chelseabfinn @percyliang . (10/n).
1
0
5
@ananyaku @2plus2make5 @leah_pierson @AndrewYNg @percyliang I joined the Stanford premed mailing list and signed up for a physician shadowing program that promised to "enhance (or even jump-start) my premedical experience"🙂.
0
0
6
@ErikJones313 blogged about our ICLR 2021 paper on how selective classification can make group disparities worse. Check it out!. And look out for more work from him -- he did this as an undergrad (!) and just started his PhD at Berkeley with @JacobSteinhardt @dawnsongtweets.🙂.
Selective classification, where models can abstain when they are unsure about a prediction, routinely improves average accuracy. Worryingly, we show that s.c. can also hurt accuracy on certain subgroups of the data. Post: 🧵.
0
1
5
@anshulkundaje @midjourney_ai Congratulations, Anshul, that's amazing!! Thank you for taking me on as a clueless CS student and teaching me some biology. Your support over the years has made a big difference to me. I'm looking forward to hearing about more cool research from the lab. :).
1
0
4
@scottgeng00's paper shows that training vision models on real data > synthetic data, so the data processing inequality lives for another day. We're still optimistic about the prospect of training on synthetic data, but more work ahead to understand when and why it might help!.
Will training on AI-generated synthetic data lead to the next frontier of vision models?🤔. Our new paper suggests NO—for now. Synthetic data doesn't magically enable generalization beyond the generator's original training set. 📜: Details below🧵(1/n).
0
1
5
We've uploaded the exact commands and hyperparameters used in our paper, as well as trained model checkpoints, to This is thanks to @tonyh_lee, who oversaw all of the experimental infrastructure and made it fully reproducible on @CodaLabWS.
1
0
4
DistShift 2021 is jointly organized with @shiorisagawa FannyYang @hsnamkoong JiashiFeng @kate_saenko_ @percyliang @slbird and @svlevine. Thank you to everyone who submitted and who helped us review. We hope to see you on Monday!.
0
0
4
#iWildCam is a fascinating dataset with lots of technical challenges, like large domain shifts and long-tailed distributions. Plus, it’s super fun to look at the animals. Check out this year's competition: it’s the same data as in WILDS, but on an even harder counting task!.
1
2
4
WILDS is a big collaboration with @shiorisagawa, who’s been instrumental in leading every aspect of the project, and a team of other amazing co-authors across 9 institutions (a small silver lining that Zoom life enabled):. (9/n).
1
0
4
Our focus is on connecting methods research with distribution shifts in real-world applications. We'll have @emwebaze speak about shifts in the context of agriculture and public health in Africa; @suchisaria on medicine; @stats_tipton on education; and @chelseabfinn on robotics.
1
0
4
We'll also hear from @aleks_madry on model debugging; Jonas Peters on statistical testing; and Masashi Sugiyama on importance weighting for transfer learning. All of our speakers have discussion slots after their talks, so come by to ask questions!.
1
0
4
@BrianFinleyEcon @causalinf @jondr44 @pedrohcgs @paulgp @jmwooldridge @percyliang IFs study how estimators change if you down-weight a point by a tiny bit; this idea was first described as the "infinitesimal jackknife". The ordinary jackknife is similar, except it removes the entire point. And it's in turn similar to the bootstrap, so they're all related!.
2
0
3
We'll end with a panel discussion on future directions on robustness to distribution shifts in different applications, featuring @AndyBeck (pathology),.@jamiemmt (algorithmic fairness), @judyfhoffman (computer vision), and @tatsu_hashimoto (NLP).
1
0
3
@hsnamkoong has done a ton of foundational work on distributionally robust optimization. This is a cool thread with a lot of info and pointers!.
A thread on distributional robustness: Performance of ML models substantially degrade on distributions different from the training distribution. For low-to-medium dimensional applications, this can be mitigated by training against worst-case losses under distribution shift. (1/n).
0
0
3
@stats_tipton Thank you for agreeing to speak at the workshop, Beth! Really looking forward to learning from your talk.
0
0
2
@abidlabs @MacAbdul9 Good question! It's an important point. We checked this where appropriate by training oracle models on data from the OOD distribution and making sure they did perform better than the standard models trained on the ID distribution; see, e.g., Tables 6, 13, 16 in Appendix E.
0
0
2
@AliHass33841763 @uwcse @GoogleAI @_beenkim Yes! I'll be looking to work with PhD students starting Fall 2023.
1
0
2
@causalinf @BrianFinleyEcon @jondr44 @pedrohcgs @paulgp @jmwooldridge @percyliang Yup, they both share similar underlying ideas. Although the jackknife is typically used in the context of estimating bias/variance, whereas influence functions are used generally in robust statistics (or, in the case of our paper, for ML interpretability).
1
0
1
@AliHass33841763 @uwcse @GoogleAI @_beenkim I'll write up an FAQ for prospective students at the end of the year, but apply for the UW CSE PhD program if you're interested!
0
0
1
@Haoxiang__Wang @shiorisagawa Thank you!! We're really glad that you're enjoying using it and we appreciate the kind note🙂Please let us know if you have any suggestions/feedback for us!.
0
0
1