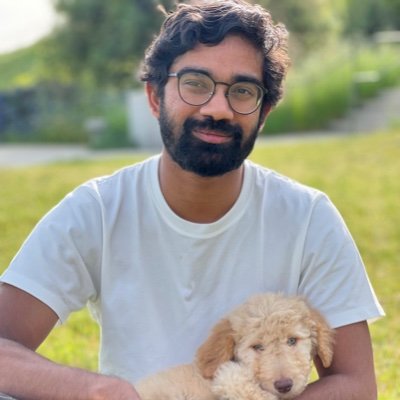
Preetum Nakkiran
@PreetumNakkiran
Followers
11K
Following
11K
Media
285
Statuses
4K
Our tutorial on diffusion & flows is out! We made every effort to simplify the math, while still being correct. Hope you enjoy! (Link below -- it's long but is split into 5 mostly-self-contained chapters). lots of fun working with @ArwenBradley @oh_that_hat @advani_madhu on this
26
269
1K
The Deep Bootstrap: Good Online Learners are Good Offline Generalizers.with @bneyshabur and @HanieSedghi at Google. We give a promising new approach to understand generalization in DL: optimization is all you need. Feat. Vision Transformers and more. 1/
7
69
329
I defended today! Thanks very much to my advisors @boazbaraktcs & Madhu Sudan, and all of my friends & collaborators along the way. In the Fall I'll be joining UCSD as a postdoc with Misha Belkin. If you're interested in my research and want to collab, do reach out!
26
3
281
It is still insane to me, from the theory side, how little architecture (and other design choices) ended up mattering. That we now use the exact same architecture for both text and vision. RIP inductive bias.
The ongoing consolidation in AI is incredible. Thread: ➡️ When I started ~decade ago vision, speech, natural language, reinforcement learning, etc. were completely separate; You couldn't read papers across areas - the approaches were completely different, often not even ML based.
11
13
234
Optimal Regularization can Mitigate Double Descent. Joint work with Prayaag Venkat, @ShamKakade6, @tengyuma. We prove in certain ridge regression settings that *optimal* L2 regularization can eliminate double descent: more data never hurts (1/n)
6
49
221
Reviewer 2 complains it has "too many ideas". @prfsanjeevarora says "gave me some new ideas". This Friday at Noon PT… come hear about the paper that reviewers don't want you to read! I'm speaking at @ml_collective's DLCT:
4
21
206
careful about overfitting to lists like this. there are many ways to do good research -- my fav papers were born out of getting "stuck in rabbit holes" that no-one else went down. .
Enjoyed visiting UC Berkeley’s Machine Learning Club yesterday, where I gave a talk on doing AI research. Slides: In the past few years I’ve worked with and observed some extremely talented researchers, and these are the trends I’ve noticed:. 1. When.
5
10
204
This paper's finally dropping on arxiv tonight. It's been an endeavor--- I've learnt a lot from many new collaborators :). One of us will tweet it out soon! Go follow @nmallinar @DeWeeseLab @Amirhesam_A @PartheP for the thread.
6
16
189
Very clean idea / paper!
@PreetumNakkiran Chatterjee's correlation coefficient for 1D RVs behaves nicely and has wonderful relationships to copula measures, HSIC, etc.
6
19
171
research is play until 6 weeks before the deadline, then research is work.
Great quote from Michael Atiyah on research and creativity (h/t @michael_nielsen). Research should be play. If your research has a roadmap, you're doing it wrong.
3
7
156
This was a very nice talk by @tomgoldsteincs, starts at 0:30.
Context for this talk was an NSF Town Hall with goal to discuss successes of deep learning especially, in light of more traditional fields. Other talks by @tomgoldsteincs @joanbruna @ukmlv, Yuejie Chi, Guy Bresler, Rina Foygel Barber at this link:.. 2/3.
2
22
154
New paper with @whybansal:."Distributional Generalization: A New Kind of Generalization". Thread 1/n. Here are some quizzes that motivate our results (vote in thread!).QUIZ 1:
2
15
144
One intuition for why Transformers have difficulty learning sequential tasks (eg parity), w/o scratchpad, is that they can only update their “internal state” in very restricted ways (as defined by Attention). In contrast to e.g RNNs, which can do essentially arbitrary updates.
There’s not enough creative exploration of the KV cache. researchers seem to ignore it as just an engineering optimization. The KV cache is the global state of the transformer model. Saving, copying, reusing kv cache state is like playing with snapshots of your brain.
2
16
135
In retrospect (knowing what we know now), the way to understand why “cold diffusion” works is via Conditional Flow Matching.
Diffusion models like #DALLE and #StableDiffusion are state of the art for image generation, yet our understanding of them is in its infancy. This thread introduces the basics of how diffusion models work, how we understand them, and why I think this understanding is broken.🧵
7
5
133
Overparameterized models are trained to be *classifiers*, but empirically behave as *conditional samplers* (cf our prior work). Here we explore learning-theoretic aspects of conditional sampling: definitions, sample complexity, relations to other types of learning, etc.
Knowledge Distillation: Bad Models Can Be Good Role Models. Gal Kaplun, Eran Malach, Preetum Nakkiran, and Shai Shalev-Shwartz
2
20
120
Since leaving academia, none of my new papers are planned for neurips/icml/iclr. The “prestige” is no longer worth the contortions required to optimize for these venues.
@kamalikac @NeurIPSConf At which point do NeurIPS, etc. require long-term teams and a long-term visibility and planning? Should it really left to overwhelmed and overworked volunteers to steer the ship one year at a time, making decisions with huge impact by reacting "the best they could"?.2/2.
3
4
119
This was a very exciting project— it changed my mental model of Transformers and compositional/OOD generalization. Check it out:
What algorithms can Transformers learn?. They can easily learn to sort lists (generalizing to longer lengths), but not to compute parity -- why? . 🚨📰 In our new paper, we show that "thinking like Transformers" can tell us a lot about which tasks they generalize on!
1
16
114
Very nice work led by Shivam Garg, @tsiprasd! .Studying in-context-learning via the simplest learning setting: linear regression. (I really like this setup -- will say more when I get a chance, but take a look the paper!).
5
16
108
excited to announce we won this year's lottery.
New paper: Modern DNNs are often well-calibrated, even though we only optimize for loss. What's going on here? The folklore answer is that we're "minimizing a proper loss", which yields calibration. But this is only true if we reach [close to] the *global optimum* loss. (1/n)
2
4
103
one thing TCS taught me is to never actually solve problems. just reduce to other problems.
@PreetumNakkiran proposed to look at the jpeg size per pixel to find the correct dimensions: works very well! . #statistics #maths
1
3
102
generally a huge fan of the philosophy "to understand a method, understand what problem it is optimally solving".
Image-to-image models have been called 'filters' since the early days of comp vision/imaging. But what does it mean to filter an image? . If we choose some set of weights and apply them to the input image, what loss/objective function does this process optimize (if any)?. 1/8
3
6
91
as a grad student, I once didn’t write any papers for 2 years. If you’re not prepared to do this, academia is for you.
As a grad student, I read each assigned reading twice before each class discussion. This often meant reading a 300 page book twice, within a week. If you’re not prepared to do this and more, I wouldn’t pursue grad school, let alone academia.
2
0
88
Our numpy implementation of RASP-L from this paper is now public: Trying to code in RASP-L is (imo) a great way to build intuition for the causal-Transformer's computational model. Challenge: write parity in RASP-L, or prove it can't be done😅.
What algorithms can Transformers learn?. They can easily learn to sort lists (generalizing to longer lengths), but not to compute parity -- why? . 🚨📰 In our new paper, we show that "thinking like Transformers" can tell us a lot about which tasks they generalize on!
1
18
88
I have yet to see a definition of “extrapolate” in ML that is precise, meaningful, and generic (ie applies to a broad range of distributions *and* learning algorithms). It’s currently “you know it when you see it”— possible to define in specific settings, but not generically.
A simple, fun example to refute the common story that ML can interpolate but not extrapolate:. Black dots are training points. Green curve is true data generating function. Blue curve is best fit. Notice how it correctly predicts far outside the training distribution!. 1/3
14
4
85
there’s so much unwritten but extremely valuable knowledge in ML: knowing which ideas actually work, and which ones sound great in the neurips papers, but don’t actually work.
As someone who just starting doing literally any machine learning this year, the most confusing part is figuring out which of the "common wisdom" is reasonable and which actually turns out to be totally outdated, and getting conflicting advice on this from different people.
3
7
87
Understanding why, in ML, we often “get much more than we asked for”* is what I consider one of the most important open questions in theory. *(we minimize the loss, we get much more, as in this paper and others).
Why does model often attend to salient words even though it's not required by the training loss? To understand this inductive bias we need to analyze the optimization trajectory🧐. Sharing our preprint "Approximating How Single Head Attention Learns" #NLProc
1
9
82
One way I like to think of this is, there are at least 3 axes in learning: sample-complexity, space-complexity (memory), and time-complexity (flops). Transformers are one point in this space, but what does the space look like? (Are they the unique optima along some dimension?etc).
From a science methods perspective, Mamba / SSM is fun. A second model challenges lots of post-hoc "why are transformers good" research to now be predictive. What if it works, but none of the circuitry is the same? Where do we go if the loss is great but it's worse?.
2
1
86
The following *very* nice piece came up in a chat re theory&applications in ML (h/t @boazbaraktcs). It was in response to a debate in TCS community. But really, most of it is a defense of "science for science's sake", and a warning about mixing scientific and industrial goals. 1/
3
8
82