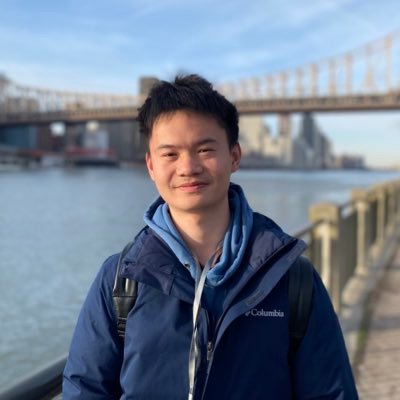
Tianyuan Zhang
@tianyuanzhang99
Followers
889
Following
2K
Media
10
Statuses
115
PhD students in@MIT, working on vision and ML. M.S. in CMU, B.S. in PKU
Boston
Joined September 2017
3D Gaussian is great, but how can you interact with it 🌹👋? Introducing #PhysDreamer: Create your own realistic interactive 3D assets from only static images! Discover how we do this below👇 🧵1/: . Website:
13
79
382
Got a chance to play pingpong in VR with this virtual agent on May, it’s so cool! .Imagine more sophisticated interactions with virtual agent in the future.
Thrilled to share our #SIGGRAPH2024 work on physics-based character animation for ping pong!🏓We show not only agent-agent matches but also human-agent interactions via VR, allowing humans to challenge our trained agents!🎮.🌐: 📜:
0
2
13
Impressive reconstruction(scene and object) results. This gives me a feeling that attention is all you need for 3D reconstruction.
Thanks @_akhaliq for promoting our work. We show that long context learning (we use up to 16k tokens) also finds its place in sparse-view reconstruction! Together with @Sai__Bi, @HaoTan5, @zexiangxu, @ambie_kk, Kalyan Sunkavalli, Nanxuan Zhao!.
1
1
11
Really impressive results. I think data driven approaches will be able to do fully inverse/forward rendering soon, including strong specular effects, hard shadows and transparencies.
Check out our recent work “Neural Gaffer: Relighting Any Object via Diffusion” 📷🌈, an end-to-end 2D relighting diffusion model that accurately relights any object in a single image under various lighting conditions. 🧵1/N:. Website:
0
0
6
@zhu_zhaocheng Feels like the biggest problem is that JAX has smaller opensource communties compared to torch on most area now.
2
0
7
Excited to share our new work on Autonomous driving:.@yuewang314, Vitor Guizilini, Yilun Wang, @zhaohang0124, @JustinMSolomon . DETR3D: 3D Object Detection from Multi-view Images via 3D-to-2D Queries . Our work allows end-to-end multi-camera 3D detection.
1
0
5
@taiyasaki @vincesitzmann Very Good demos. But feels like, to get better results with fewer images, LFN needs more modeling on the scene, thus drops one big advantages over NeRF: no explicit modelling/contraints of the rendering process. -- Using depth reg assumes near Lambertian scene and no occlusion.
1
0
3
So cool.
check out my latest trailer, Sand, .crafted using my favorite ai tools:. @midjourney for image generation .@runwayml gen-3 for video creation .@VideoleapApp for seamless editing. making videos like this feels like magic
0
1
6
@james_y_zou @mertyuksekgonul @federicobianchy @ria_kalluri @jurafsky Exciting work! I feel similar problem also occurs on stable-diffusion. Where generated images hardly follow the composition of the text prompt.
1
0
3
Work done with @Koven_Yu, @ChrisWu6080, Brandon Y. Feng, Changxi Zheng, @Jimantha, @jiajunwu_cs, and Bill Freeman. 5/5.
0
0
2
@Aaronf_hd @janusch_patas I also use metashapes and it’s way faster and robust. But i don’t know why. One general assumption is that heavy engineering is very important for implementing algorithm with long pipeline like SFM, and metashapes just have the money to engineering the system quite well.
1
0
1
@dr_cintas So cool! From these demo videos, the speed of generative feels to be “real time”(few seconds). That’s quite impressive if they are using diffusion models on a cpu machine.
0
0
1
@liangchensong @taiyasaki @vincesitzmann Thanks for the replying. I think your work is a good one, it shows a good balance between more prior and being versatile. Looking forward to your future works!.
0
0
1
@Xianbao_QIAN Good question! It's slow. We mentioned the speed in the final Limitation section of the paper. Our implementation takes 1 min of a V100 GPU to produce 1 second of the simulated video.
5
0
1
@rrika9 remind me of the range analysis paper on sdf: Spelunking the Deep: Guaranteed Queries on General Neural Implicit Surfaces via Range Analysis.
0
0
1
We introduce #PhysDreamer. The key idea is to distill the object dynamics priors learned by a video generation model to estimate the physical materials of static 3D objects. 3/N
1
1
1