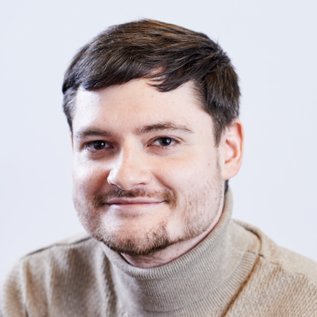
Patrick Schwab
@schwabpa
Followers
7K
Following
29K
Media
220
Statuses
1K
Senior Director Machine Learning & AI @GSK. Prev: ML @Roche, PhD @ETH. ML for Drug Discovery and Health.
Zurich, Switzerland
Joined July 2013
You couldn't make it to #NeurIPS2022 this year?. Nothing to worry - I curated a summary for you below focussing on key papers, presentations and workshops in the buzzing space of ML in Biology and Healthcare 👇
23
153
791
The Machine Learning for Drug Discovery workshop is back for #iclr2023 ! . Featuring fantastic speakers @CarolineUhler, @mmbronstein, @OkkoClev, @lizbwood, @fabian_theis & @RGBLabMIT. We are calling for papers & are opening a challenge. See you there!.
4
97
438
Could not echo this message more: if you are excited about making a difference at scale, the intersection of engineering and bio/medicine is the place to be. However, do go into it with open eyes:.- be ready (and ideally eager) to spend your whole career in this area. Solutions.
Seems like a consensus is forming amongst many smart people! . This is Jensen Huang, CEO of $NVDA. Basically WATCH OUT for the impending biotech BOOM 💥
14
44
364
Unable to keep up with the deluge of amazing work happening in ML for Biology and Health at NeurIPS this year?. We've got you covered with a concise summary of #NeurIPS2023 content focussed at the exciting intersection of Biology, Health and AI!. thread 👇
5
63
331
For all its flaws, I would argue the biotech industry is still *the* single best place to work today with a tech background. 1- Intellectually, you get to work on some of the hardest problems of our time and they won’t be solved anytime soon. 2- The field is undergoing a.
Why is biotech such a sad industry? . They make medicine, it output feels 10x more important than automation and info-plumbing (aka the tech industry) and requires deeper knowledge/training -- and yet, low salaries, perpetual layoffs, very few founder CEOs, bad vibes all around.
14
43
325
Reality check. - novelty: 0.- creativity: 0.- likelihood to cure all cancer: 0%. Information retrieval does not solve scientific discovery
Sam Altman says AI will someday be able to cure cancer in collaboration with humans by suggesting wet lab experiments to do and then thinking about the results
20
24
260
The inevitable journey of any AI in drug discovery practitioner
Super thought-provoking piece by @AndrewE_Dunn on Isomorphic. The whole company is a big middle finger to the almost universally held dogma in TechBio that data is the key bottleneck.
4
19
210
If you work in BioAI and you live in constant fear of being scooped, consider that you may be working on the wrong problems. Or, in other words: Drug discovery consists of 1000s of steps, PDB does not answer all of them.
TBH, it has got to be stressful working on AlphaFold 3 reproductions -. We have seen, what, 4 of them come out within days of one-another. But its awesome for the community as we get more open source tools to play with, too :).
6
17
165
Feeling like you missed out on all the amazing progress at the intersection of Health & Bio presented at #ICML2023 in Honolulu?. We've got you covered with a curated, whirlwind review of all the exciting science at the emerging interface of ML/AI, health and biology . 👇thread
3
28
144
Perhaps controversial (?) take: computational models that faithfully represent biology are guaranteed to be not interpretable. Biology consists of elements that are . - redundant .- non-modular.- probabilistic.- multi-functional.- time-resolved.- multi-scale. None of these.
Cells are not black boxes. They are interpretable, because they are made of atoms. With sufficient research (and effort), we can figure out exactly how they work. "Black box" predictive models are useful, insofar as they are accurate. But the goal of the coming decades should
12
17
131
I would not recommend to make the below your mantra for AI in drug discovery. - Metadata is key - no amount or mixture of data without enough information about the context in which the data were generated will give you generalizable insights, nor will you have any hope to.
From everything I see day to day, deep learning for drug design has exactly the same characteristics as other DL-successful domains: .- dataset design and mixture is key.- scalable and stable architectures and losses trump all.- end-to-end is better, just follow the gradient.
10
15
108
Dismissing the growing attention on AI in biotech as hype is short-sighted and misses critical nuance . AI in biotech is in a curious state of Heisenbergian superposition, and is simultaneously over- and underhyped. Overhyped: .Superficial use of AI while addressing traditional.
ai in biotech is incredibly overhyped. biotech companies live and die by concrete assets generated from carefully selected disease models with rational biological hypotheses. engineering efforts are better spent centralizing and structuring experimental data for basic analysis.
5
11
112
Machine Learning for Drug Discovery (MLDD) is coming back! . With outstanding speakers: .@lucycolwell37, @UWproteindesign, @ThoreG, @KrishnaswamyLab, @shantanuXsingh & @Avsecz. We meet on Monday, May 6 (before #iclr2024) in Vienna. See you there! .
1
16
105
Just one week until the Machine Learning for Drug Discovery (MLDD) event in Vienna, Austria!. With a stellar line-up of speakers: .@lucycolwell37, @UWproteindesign, @deboramarks, @ThoreG, @KrishnaswamyLab , @shantanuXsingh & @Avsecz . guiding us through the most exciting
5
18
103
Perhaps a contrarian view but there are some major holes in the "scaled data-generation is all you need" hypothesis too:. 1) observational data without perturbations in general does not enable inference of causal relationships (e.g., if you are generating unperturbed scRNAseq.
Another nice AI bio piece by @AndrewE_Dunn, interviewing @demishassabis this time. It is interesting to see a second cycle now of in silico emphasis > data, where the first cycle was shifted to differentiated data approaches. AlphaFold is exciting and I can think of ways.
5
8
87
@rbhar90 I concur. I am surprised how even some of our smartest seem to fall victim to the above fallacy - what do you think leads to the pervasive overestimation of capabilities of AI? . (this seems to happen with every step-change in progress; people were making similar claims when RL.
4
4
78
Lots of insightful nuggets in the recent @endpts interview with @DaphneKoller:. Few problems in AI in bio are compute-bound today. In contrast to more data-rich AI domains like imaging and language, there are few problems in AI in biology where the active binding constraint is.
5
12
71
We recently evaluated the risk of sharing de-identified open health data, and found little evidence of harm relative to the growing issue of data breaches that affect millions. Great collaboration with Leo Celi and team.@MITCriticalData @GSK @harvardmed.
2
11
57
In vitro experiments, using e.g. CRISPR, have become essential in early drug discovery. However, with billions of potential experimental designs, the search space is vast and selecting the right experiment to conduct can be difficult. In #ICLR2022 work we introduce GeneDisco. 👇
1
10
56
Interesting contrast between former AI lab employees, who appear to uniformly believe it is easy to build another LLM company from scratch, and investors that believe these companies will have an enduring moat. I wouldn’t bet against the people closer to the technology on this.
BREAKING NEWS 🔥 Mira Murati, former OpenAI CTO to raise $100M for new AI startup. The company will train proprietary models to build AI products. Barret Zoph from OpenAI is expected to join the company too.
6
4
56
Aggregated trial data paints a more differentiated picture - the overwhelming majority of clinical studies are started in the EU/US. - the overall mix appears more or less stable post COVID. - the, likely economically-driven, drop in studies being started since 2021 is *larger*
In biotech, China now ahead in ADCs, bispecific Ab’s, T cell engagers, and traditional small mlc’s . US still slightly ahead on cell and gene therapy, gene editing, and more exotic small molecules (glues, covalents, degraders, etc) . China clinical dev timelines 50-100% faster.
3
7
55
At the excellent @icml_compbio workshop, @mmbronstein (@UniofOxford) gave a captivating talk on the enormous potential of geometric ML for molecules, covering applications from molecular impainting, fragment based molecular design and protein function prediction.
2
6
48
In a world of exponentially expanding data, you can find evidence to the contrary for every idea. In drug discovery - where most ideas fail - this means the consensus will generally be opposed to all unproven ideas. You add value where you have conviction that goes against.
Pharma R&D head to his BD team: “We look at many opportunities and can find a reason to say no to every one of them. If we always say no, we will be right almost all the time but we won’t make any money”.
1
7
49
Amazingly rich insights in the recent interview with @SchreiberStuart (@arenabioworks) and @RLCscienceboss (@endpts):. Many inefficiencies remain to be addressed in going from fundamental research to medicines available in the clinic. Any researcher with an interest in having
4
4
43
This all sounds very cool until you realize absolutely every disease X has been connected with every condition Y in literature. We love to romanticize simple ideas about scientific discovery but this is not a systematically exploitable strategy. Using the example from the.
making scientific discoveries simply by connecting information already existing in the public domain
6
2
44
Imagine the things we could do if we brought this precision mindset to medicine.
From early design to volume manufacturing, our computational lithography software enables chipmakers to optimize the chip patterning process. Layer by layer, chip by chip, wafer by wafer, computational lithography is helping push microchip technology to new limits.
3
3
44
Stellar keynote by Aviv Regev on how ML is being used in Drug Discovery @genentech. Covers gems like lab-in-the-loop for mechanistic discovery, small molecules, large molecules and personalised cancer vaccines. A great overview on the field. slides:
2
7
39
This is perhaps the most common misunderstanding in working with language models - to assume they "think" like humans. @paulg expects ChatGPT counts occurrences of different questions people raise, and expects an answer based on this information. What actually happens: The.
6
3
36
MLDD just got even more exciting!. @deboramarks is joining our exceptional group of speakers!. Ready for a tour of the world of ML for DD - protein design, function & language models, cell imaging, single cell and genomics?. When? Mon May 6 (before ICLR).Where? Vienna Austria
1
2
32
The closest thing to arbitrage in drug discovery is to use tools that have just been figured out on problems where they are clearly applicable. No scientific glory, no potential for fancy publications - hence fewer people looking at it than warranted by the potential.
There aren't enough smart people in biology doing something boring. new argument piece . i often meet a lot of founders in biology pursuing really crazy, revolutionary ideas. i love them! but i wish there were more people pursuing the boring ideas. 🧵.
2
1
33
A positive side benefit of removing gene names would be that bioinformaticians wouldn't have to spend half of their day normalizing gene names anymore. :-).
A central mistake in biology was to name genes. This over-simplification made reconciling what is happening on the molecular level a mess - it's not rare to find reports of opposite mechanisms in different contexts, claimed involvement in dozens if not hundreds of different
7
5
33
A simple litmus test to debunk grande AI over-claims like the below is to ask yourself if the data the models are trained on even contain the information required. Could you possibly build a physical world model from predicting the next frame in a video sequence like the below?.
If you think OpenAI Sora is a creative toy like DALLE, . think again. Sora is a data-driven physics engine. It is a simulation of many worlds, real or fantastical. The simulator learns intricate rendering, "intuitive" physics, long-horizon reasoning, and semantic grounding, all
10
4
29
Giving models an understanding of what they do not know, is for many decision-making applications as important as providing accurate predictions. E Candès @Stanford gave a broad introduction to conformal prediction with quantile regression to filter out low confidence predictions
1
3
29
For an AI researcher, it may be shocking to learn that e.g. in the field of medicine we most often simply don’t know what works and what doesn’t. We rarely know what practices systematically lead to better outcomes, how good we are at diagnosing, whether we should be testing.
As we hit 20’000+ NeurIPS submissions today, I suspect an under-appreciated reason why almost everyone with ambition is gravitating to the AI/ML field is because the field is radically empiricist - in ML research ‘good’ is what works quantifiably. In contrast to more structured.
0
4
28
On second thought, "building platforms before making medicines" is such a fundamental and common mistake in the field that it could be the AI-driven biotech equivalent of "not talking to users" in the software domain.
A central challenge of AI-driven biotech is that in drug discovery a fully validated medicine is worth a million times more than a million early-stage ideas that only have little validation. This is unfortunately at odds with the AI domain where we almost always attempt to
0
4
28
Viruses mutating in response to immune pressure is one of the key challenges in effectively responding to pandemics. In recent work Thadani @sarahgurev @NotinPascal et al (@DeboraMarksLab) developed EVEscape to predict viral escape from sequences, biophyiscal and structure. 👇
1
3
28
@educationpalmer @Google @Apple @IBM Unsurprising. For a software engineer, a strong github portfolio, for example, is a much stronger signal than any degree.
0
0
23
Starting off with Keynote presentations: . Back prop has become the workhorse in ML-.@geoffreyhinton challenges the community to rethink learning introducing the Forward-Forward Algorithm that are trained to have high goodness on positive and low goodness on negative samples.
1
6
26
One thing that Jensen Huang and @nvidia do not get enough credit for is just how incredibly prescient they were in betting on the role of GPUs in AI. They noticed the early signs in leading AI research groups starting to use GPUs for machine learning, including its contribution
2
0
20
The Image2Omics preprint with this & more is available at:. Amazing collaboration with @cuong_qnguyen, R Mehrizi, @arashmeh, @MBantscheff, @maryalegro, B Carbone, C Fishwick, H Keles, S Sanford, Yi Zhao and the talented dry and wetlab scientists @GSK!.
1
4
24
In the AI for Science workshop, David Baker @UWproteindesign gives us a whirlwind tour of the exciting progress in de novo protein design with ML incl the first approved de-novo designed medicine, amyloid binding, protein/NA complexes and RFdiffusion for unconditional generation
1
6
22
More good news today: Our paper on reducing false alarms in critical care with very few labels got accepted to #icml2018 @icmlconf! Many thanks to our collaborators at @Unispital_USZ @ETH_en @uzh_news_en . Paper link:
1
6
22
Saving 4 hours of sleep per night sounds great. What could possibly go wrong?. Cardiovascular disease (stroke, heart attack, . ) risk +50%. whatever biological relationship we think is simple probably is everything but.
There are people who genetically only need 4-6 hrs of sleep per night. They also "tend to be more optimistic, more energetic and better multitaskers. They also have a higher pain threshold, don’t suffer from jet lag and some researchers believe they may even live longer.". We.
4
1
22
Surprisingly, the church of ‘scale is all you need’ still has many acolytes - ‘situational awareness’ is only a few months old. ‘Emergence’ of course doesn’t exist and when you train a model to recapitulate associations in your data, you, naturally, get exactly what you trained.
People have been rewriting history and saying that "everyone has always believed that LLMs alone wouldn't be AGI and that extensive scaffolding around them would be necessary". No, throughout most of 2023 (the "sparks of AGI" era) the mainstream bay area belief was that LLMs were.
1
6
21
Exciting news for AI-driven drug discovery - Xaira, another entrant in the $100m+ heavyweight league emerges from stealth with, among others, protein design pioneer David Baker (@UWproteindesign). What they shared below points towards bringing together what emerged over the last.
Among the head-turning details:. ex-Stanford prez Marc Tessier-Lavigne returns to biotech as CEO. investors include ARCH, Foresite, NEA, F-Prime, Sequoia, Lux. board includes Carolyn Bertozzi, ex- J&J CEO Alex Gorsky, former FDA head Scott Gottlieb.
0
2
21
@mo_lotfollahi The community should scrutinise bold claims around "foundation models" more. Some of these models claim to be good at tasks that are well established (e.g. GRN inference, perturbation prediction) but do not compare to existing work on comparable benchmarks.
1
0
19
@stefanAbauer @CIFAR_News @KTHuniversity presented a wealth of recent research on real-world uses of causal inference including single-cell gene network inference, learning neural causal models from perturbational data, and optimal experimental design for causal discovery.
1
2
19
Causality in biology leader @CarolineUhler @MIT makes the case for the new methods needed to truly move towards causality in ML and introduces us to an impactful application to cancer immunotherapy for which a $USD 50'000 challenge has been opened:
2
2
18
Claims of progress towards AGI via emergence are greatly exaggerated. LLMs perform web-scale semantic information retrieval. Common benchmarks feign progress because of increasing test-set leakage and manual fine-tuning via RLHF. This represents intelligence only if one.
My mental model of an LLM is an interpolative vector database. Larger LLMs correspond to bigger databases. The vector embeddings allow for interpolative behavior to generalize to some degree due to rich embeddings. Given this model, I am trying to understand the scientific.
1
0
15
@rbhar90 I would actually dare to predict with high confidence that next word prediction would not necessarily be part of a potential future true general intelligent system (in fact any system in which we would classify intelligence not in the sense of information retrieval).
2
0
18
Causality is at the core of key problems in healthcare and is receiving more attn at NeurIPS:. In the causalML for real world impact workshop, causality pioneer Peter Spirtes @CarnegieMellon (of PC algo fame) outlines challenges, limitations and paths forward for causal inference
1
1
17
After releasing their leading protein language model ProGen last year, @thisismadani and team @ProfluentBio now present a breakthrough in the application of bioLMs to designing CRISPR-like molecules capable of gene editing with high precision. Moreover, they release the result.
Can AI rewrite our human genome? ⌨️🧬. Today, we announce the successful editing of DNA in human cells with gene editors fully designed with AI. Not only that, we've decided to freely release the molecules under the @ProfluentBio OpenCRISPR initiative. Lots to unpack👇
0
3
16
A month ago @leopoldasch warned of a coming "Intelligence Explosion" based on projected automation of AI research, and claimed awareness of these scenarios is a matter of international security. But how realistic were the scenarios?. To find out, I used napkin math to calculate
3
0
16
@DdelAlamo Generally, one should be skeptical when reported errors are lower than the experimental uncertainty. :-).
0
0
16
Causal explanation (CXPlain) models that learn to explain any other machine-learning model to be presented at #NeurIPS2019. Includes experiments on MNIST, ImageNet and Twitter sentiment analysis, and we show how to quantify estimation uncertainty. Link:
1
4
14