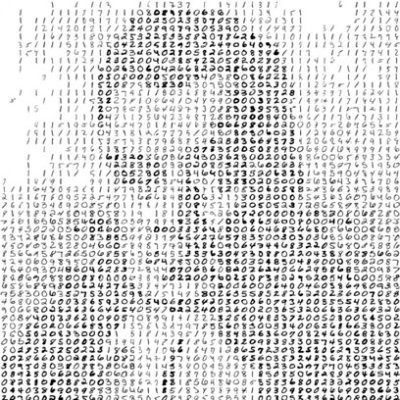
Griffiths Computational Cognitive Science Lab
@cocosci_lab
Followers
5K
Following
84
Media
9
Statuses
160
Tom Griffiths' Computational Cognitive Science Lab. Studying the computational problems human minds have to solve.
Princeton, NJ
Joined August 2020
Habituation and comparisons can result in depression, materialism, and overconsumption. Why are these disruptive features even part of human cognition? .New paper with Rachit Dubey and Peter Dayan in @PLOSCompBiol provides a RL perspective on this question.
2
36
143
In this new paper we apply a cognitive science approach to large language models like GPT-4. Focusing on the problem LLMs were trained to solve - predicting the next word - reveals a lot about their behavior. Simple tasks can result in errors when they push against this training.
🤖🧠NEW PAPER🧠🤖. Language models are so broadly useful that it's easy to forget what they are: next-word prediction systems. Remembering this fact reveals surprising behavioral patterns: 🔥Embers of Autoregression🔥 (counterpart to "Sparks of AGI"). 1/8
0
13
69
Four years in the making, this paper presents a new and detailed picture of biases in human spatial memory. By having people repeatedly reconstruct a location on an image from memory, we were able to clearly see which locations attracted people's reconstructions (and why!).
We are excited to share our recent publication in PNAS: Serial reproduction reveals the geometry of visuospatial representations by @ThomasALanglois,* @norijacoby,* @suchow, & @cocosci_lab @CompAudition @MPI_ae @columbiacss @PNASNews @maxplanckpress (1/8).
0
15
64
Being able to collect large amounts of behavioral data allows us to revisit classic results in cognitive psychology established with simple laboratory stimuli to see whether they hold with more naturalistic stimuli. Shepard's law is just as beautiful for real images.
1/6 Happy to share that our paper “The universal law of generalization holds for naturalistic stimuli” is out in the March issue of Journal of Experimental Psychology: General as the Editor’s Choice article!
0
11
62
How should you design a mind? New paper with @SmithaMilli and @FalkLieder shows agents who rationally select a system for a decision benefit from having more than one system but returns diminish rapidly. A new view of dual-process (fast and slow) theories.
1
22
55
Our new paper uses neural networks to invert cognitive models. We use a model to generate large amounts of simulated data relating an internal state to behavior. These data can be used to train a neural network that learns the inverse mapping, predicting people's internal states.
👀🍟Now out in Cognitive Science 😋🤖. We present a new approach that combines cognitive models and neural networks to predict latent preferences.
0
9
53
Our new paper uses an experimental simulation of social networks to demonstrate they magnify biases in decision-making, then shows an algorithm from statistics (importance sampling!) can give the benefits of sharing information without increasing biases. Ironically sharing here.
Four years in the making, my main PhD work is in Nature Human Behaviour!. Decades of work has shown both benefits and costs to group decision-making. Can we restructure social networking algorithms so there are fewer costs and more benefits?. Paper: 1/10
0
4
45
We analyzed online chess games to see whether people make intelligent decisions about how long to think. We found that chess players spend more time thinking in situations where planning is more valuable. You can decide if it is worth your time to learn more!.
New preprint We examined how chess players spent limited time available for thinking in over 12 million online games and found this tracks value of applying planning computations. w/ Dan Acosta-Kane (co-lead) @basvanopheusden @marcelomattar @cocosci_lab.
1
5
42
New paper explores the implications of alignment of representations between humans and machines for the performance of those machines when learning from small amounts of data. Being aligned with human representations helps, but so does being strongly misaligned. we explain why!.
🧵 Excited to share another new paper with @cocosci_lab, accepted as a spotlight at #NeurIPS2023! 🎉 We delve into the intriguing intersection of AI and human cognition, exploring how alignment with human representations impacts few-shot learning tasks.🧠🤖🎓 Let's unpack this!👇
0
5
41
In this new preprint we use data from millions of online chess games to show that mechanisms of reinforcement learning and social learning that are normally studied in the lab influence complex decisions that are learned over months, such as how to start a game of chess.
New preprint! The first from my postdoc, on work with @basvanopheusden @evanrussek and @cocosci_lab. We use a data set of over two million decisions to show that people employ sophisticated learning algorithms in naturalistic strategic behavior. More in 🧵
0
14
41
(5/5) Thanks to the many contributors to the book! @NickJChater @MITCoCoSci @YuilleAlan @mark_ho_ @RajaMarjieh @norijacoby @ThomasALanglois @jingxulvscience @EdVul @ermgrant @callfredaway @FalkLieder @TomerUllman @jhamrick @tobigerstenberg @spiantado @noahdgoodman @E_Bonawitz.
5
1
42
New paper! We use the implicit inductive bias of gradient descent to efficiently implement Bayesian filtering in a way that can scale using modern machine learning tools.
Bayesian filtering can be done efficiently and effectively if framed as optimization over a time-varying objective. Below, we use adaptive optimizers, like Adam, to internalize the inductive bias of Bayesian filtering equations. Instead of turning the crank on Bayes’ rule, we
0
6
40
New paper! Nudging has been a popular way to guide people's decisions, but it doesn't always work. We present a framework that allows us to predict when nudges will be effective and test them in a controlled setting. We then use this framework to optimize for effective nudges.
Our paper on optimal nudging is out in Psych Review! We use resource-rational analysis to formalize nudges and predict their effects. We also show how to use this approach to automatically construct *optimal* nudges that best improve choice. With @callfredaway and @cocosci_lab
0
7
39
New preprint explores how classic ideas in cognitive science - production systems and cognitive architectures - provide a framework for designing cognitive agents based on large language models. Connections to Australian marsupials purely coincidental.
Language Agents are cool & fast-moving, but no systematic way to understand & design them. So we use classical CogSci & AI insights to propose Cognitive Architectures for Language Agents (🐨CoALA)!. w/ great @tedsumers @karthik_r_n @cocosci_lab .(1/6)
3
4
38
Embeddings are often analyzed to see what neural networks represent about the world. This new preprint explores what they *should* represent, showing that autoregressive models (like LLMs) should (and do) embed predictive sufficient statistics, including Bayesian posteriors.
🤖📃New preprint: What should embeddings embed? . Embeddings from large language models (LLMs) capture aspects of the syntax and semantics of language. In a new paper, we develop a theory of what aspects of the input embeddings should represent. Link:
0
4
39
Our new preprint shows that using chain of thought prompting can result in a significant drop in the performance of AI models on tasks where getting people to express their thoughts reduces human performance.
Is encouraging LLMs to reason through a task always beneficial?🤔. NO🛑- inspired by when verbal thinking makes humans worse at tasks, we predict when CoT impairs LLMs & find 3 types of failure cases. In one OpenAI o1 preview accuracy drops 36.3% compared to GPT-4o zero-shot!😱
0
7
35
In this fun new paper we use a programming game to explore people's preferences for the structure of hierarchical plans.
Human behavior is hierarchically structured. But what determines *which* hierarchies people use? In a preprint, we run an experiment where people create programs that correspond to hierarchies, finding that people prefer structures with more reuse. 1/7
0
4
31
New preprint showing that pretraining language models on arithmetic results in surprisingly good performance in predicting human decisions. This kind of focused pretraining can be a useful tool for figuring out why LLMs predict aspects of human behavior.
Large language models show promise as cognitive models. The behaviors they produce often mirror human behaviors, suggesting we might gain insight into human cognition by studying LLMs. But why do LLMs behave like humans at all?🤔🧵
0
3
29
New preprint shows large language models inaccurately predict humans will make rational decisions. Using chain of thought prompts result in predictions based on expected value. However, this assumption of rationality aligns with how humans make inferences from others' decisions.
New preprint: LLMs assume people are more rational than we really are 😮🤖. Applies to simulating decisions, predicting people’s actions, and making inferences from others’ decisions. link: [1/4].
0
0
29
New preprint offers a resource-rational take on the classic problem of choosing subgoals: select subgoals to reduce the cost of planning. Analyzing many graphs representing planning tasks shows this approach can explain why existing heuristics work and capture human subgoals.
✨Preprint✨ w/ @mark_ho_, @callfredaway, @nathanieldaw, & Tom Griffiths: TLDR: How do people decompose goals into subgoals? We ran a large-scale experiment to test how the computational cost of planning drives subgoal choice. More in 🧵
0
5
27
New preprint translates a method cognitive scientists have used to elicit human priors into a method for studying the implicit knowledge used by Large Language Models.
Large language models implicitly draw on extensive world knowledge in order to predict the next word in text – the equivalent of a Bayesian prior distribution. But how can we reveal this knowledge? 🧵
0
2
28
Exciting new preprint combining Bayes and neural networks via metalearning! The key idea is distilling a prior distribution from a symbolic Bayesian model to support faster learning by a neural network. The application here is language, but this is a very general approach.
🤖🧠NEW PAPER🧠🤖. Bayesian models can learn rapidly. Neural networks can handle messy, naturalistic data. How can we combine these strengths?. Our answer: Use meta-learning to distill Bayesian priors into a neural network!. Paper: 1/n
0
2
26
New preprint uses methods from psychology to explore implicit biases in large language models. Using simple prompts that probe associations between social categories, models that have been trained to be explicitly unbiased show systematic biases.
🚨 New Preprint 🚨.OpenAI says that the new GPT-4o model is carefully designed and evaluated to be safe and unbiased. But implicit biases often run deep and are harder to detect and remove than surface-level biases. In our preprint, we ask, are GPT-4o and other LLMs really
1
2
27
How should you take into account what other people think? Our new paper shows that a simple Bayesian model can capture how people make inferences from aggregated opinions.
My latest paper is out at Psych Sci❗️ .w/ @TaniaLombrozo & @cocosci_lab. We built a Bayesian model that captures people's inferences from opinions* in a game-show paradigm. *e.g., if 12 people think 'X' is True, and 2 think 'X' is False, P(X=T) = 81%.
0
6
25
How can algorithmic causality help us understand transfer in RL?.@mmmbchang will present "Modularity in RL via alg independence" in #ICLR2021 ws:.Generalization: 1pm PT: Learning to learn: 8:40am PT: w/ @SKaushi16236143, @svlevine
0
5
24
New paper uses a novel theoretical framework and a large behavioral experiment to tease apart predictions of models of task decomposition (ie. subgoal selection). Key idea is formulating the problem of choosing subgoals in terms of reducing the computational cost of planning.
I'm excited to share that my paper on resource-rational task decomposition has been published in PLOS Computational Biology! 🎉🥳 . TLDR: People break large tasks down into smaller ones by balancing efficiency and the computational cost of planning.
0
4
23
How do people teach abstractions? Our new preprint shows that people tend to focus on even simpler examples than Bayesian models of teaching suggest.
🚨New preprint!🚨Excited to share my 1st PhD project with @BonanZhao @cocosci_lab @natvelali.Teaching is a powerful way to pass on "tools for thought" to solve new problems. How well do existing teaching models of teaching capture this process?.Link:
0
1
23
We are looking for a new lab manager, shared with the Concepts and Cognition Lab of @TaniaLombrozo. Apply here:
1
20
23
Individuals making discoveries isn't enough to produce progress: other people need to learn from and build on those discoveries. In this new paper we show how being able to chose who to learn from plays an important role in the cultural evolution of complex cognitive algorithms.
Over the last five years @cocosci_lab we've been building the capacity to conduct larger and more structured simulations of cultural evolution in the lab. The results of our latest experiment (with @basvanopheusden & @tedsumers) were just published.
1
3
21
This paper will be of interest to people studying cultural evolution as well as reinforcement learning: in addition to identifying what makes some algorithms transfer better to new tasks, we show that decomposing tasks across members of a society is one way to support transfer.
Does modularity improve transfer efficiency?. In our ICML’21 paper (long oral), we analyze the causal structure of credit assignment from the lens of algorithmic information theory to tackle this question w/@SKaushi16236143, @svlevine, @cocosci_lab.1/N
0
7
20
Thinking about distributed systems isn't just a useful way to understand human collaboration, it might give you a new argument (or start one) next time you don't help clean up after dinner.
🚨New preprint!🚨 My first first-author project with @RouseTurner @natvelali @cocosci_lab. How do people evaluate idle collaborators who don’t help out during group tasks?. TL;DR: Sometimes it’s okay not to help with the dishes 🧵👇. Link:
0
0
21
Videos from the CogSci workshop on Scaling Cognitive Science are now online! Enjoy talks by Tom Griffiths @jkhartshorne @_magrawal Andrea Simenstad @the_zooniverse @suchow @todd_gureckis @joshuacpeterson Lauren Rutter and James Houghton
1
2
22
New paper using some classic ideas from cognitive science to improve problem-solving by large language models!.
Still use ⛓️Chain-of-Thought (CoT) for all your prompting? May be underutilizing LLM capabilities🤠. Introducing 🌲Tree-of-Thought (ToT), a framework to unleash complex & general problem solving with LLMs, through a deliberate ‘System 2’ tree search.
0
2
20
Our new preprint "Cognitive science as a source of forward and inverse models of human decisions for robotics and control" is now posted!.
Now in press at Annual Review of Control, Robotics, and Autonomous Systems! Tom Griffiths (@cocosci_lab) and I take a whirlwind tour of research on human decision-making and theory of mind in relation to control and robotics 🤔 💭 🤖 1/5
0
0
18
Is the Dunning-Kruger effect just accurate Bayesian estimation of ability? In a new paper @racheljansen fit a rational model to metacognitive judgments in two large studies, revealing the form of the relationship between performance and self-assessment.
1
4
20
See our paper w/ @mmmbchang, @SKaushi16236143, @svlevine, NeurIPS DeepRL workshop Dec 11: causal analysis on the *structure of an RL alg*, towards formalizing modular transfer in RL.Poster: Paper: Video:
0
5
16
If you’ve ever wondered what's the fastest way to get to a supermarket checkout, whether organizations need hierarchical structure, or how to get a group of people to agree, you might enjoy this new @audible_com series by @brianchristian and Tom Griffiths
1
6
17
New preprint applies rational metareasoning to large language models, achieving similar performance while generating significantly fewer chain-of-thought tokens.
🚀 Excited about LLM reasoning but hate how much o1 costs? What if LLMs could think more like humans, using reasoning only when necessary? Introducing our new paper: Rational Metareasoning for LLMs! 🧠✨.
0
0
17
This was a fun collaboration that started with @AnnieDuke asking whether optimal stopping offered insight into when to quit and quickly turned into a lot of math and data collection. The answer: it can, but we had to come up with a new optimal stopping paradigm to get there.
I have a new preprint out with the fabulous @cocosci_lab on whether people make optimal decisions about when to persist and when to quit.
0
1
16
Do people remember better as a group, or by themselves? We used crowdsourcing to turn an agent-based model of collaborative memory into a large-scale behavioral experiment in our new paper with @vaelgates @suchow.
1
6
16
New paper! Cognitive science has a long tradition of thinking about the tradeoffs inherent in language, which can give us useful tools for understanding large language models. While we want our models to be honest and helpful, sometimes those goals conflict.
Honesty and helpfulness are two central goals of LLMs. But what happens when they are in conflict with one another? 😳. We investigate trade-offs LLMs make, which values they prioritize, and how RLHF and Chain-of-Thought influence these trade-offs: [1/3].
0
0
15
New paper explores the factors contributing to the success of chain-of-thought reasoning in large language models using a fun and carefully controlled task: solving shift ciphers.
🤖 NEW PAPER 🤖. Chain-of-thought reasoning (CoT) can dramatically improve LLM performance. Q: But what *type* of reasoning do LLMs use when performing CoT? Is it genuine reasoning, or is it driven by shallow heuristics like memorization?. A: Both!. 🔗 1/n
0
2
15
New work from the lab on meta-learning.
Excited to share a new preprint w/ great collaborators, Ishita Dasgupta, Jon Cohen, Nathaniel Daw (@nathanieldaw ), and Tom Griffiths( @cocosci_lab) on meta-learning in humans and artificial agents!
0
1
13
People are good at finding simple representations that help them solve planning problems. In this new paper, we present a framework for understanding this by identifying optimal construals of planning problems that are simple but yield good plans.
1/ I'm excited to share our new paper, now in @Nature!. Paper: PDF: Summary 🧵:. We present a new theory of problem simplification to answer an old question in cognitive science and AI:. How do we represent problems when planning?.
0
2
11
Check out Fred's paper, which derives from first principles a new model of what people look at when making a decision: eye movements are predicted by the optimal policy for sampling to determine the item with highest expected value.
I'm very excited to announce my first first-author journal paper: "Fixation patterns in simple choice reflect optimal information sampling" @PLOSCompBiol w/ Antonio Rangel and Tom Griffiths @cocosci_lab 1/.
0
0
11
Our new paper presents a computational model of functional fixedness and a simple empirical paradigm for testing it, building on work by @mark_ho_ on efficient task construals in planning. Getting used to one construal of the world can cause problems!.
Excited to share a new paper just accepted at Psych Science 🎉. Preprint: We look at one of my all time favorite cognitive biases:. ✨💭 Functional Fixedness 💭✨. Have you heard the idiom “To a person with a hammer, everything looks like a nail”?. A 🧵
0
2
10
What can a large web-based game of “telephone” tell us about how people recognize spoken words? Find out in our new article in Cognition led by @StephanMeylan (doi: preprint: .
0
4
10
In this new paper with @RajaMarjieh @sucholutsky @ThomasALanglois and @norijacoby (@CompAudition) we show how a classic cognitive science experiment can help us understand the diffusion models used in popular AI image generation systems.
Excited to share our new work which identifies a correspondence between diffusion models and serial reproduction, a classic paradigm in cognitive science similar to the telephone game! We use this to provide a new understanding of why diffusion models work so well. 1/n
0
1
11
Congratulations to our current lab manager @maya_malaviya who is heading off to start a PhD with @mark_ho_ !.
0
0
10
We spoke not just with computer scientists (including @red_abebe @JohnDCook @julie_a_shah @heidiann360 @eric_brewer @MilindTambe_AI),.but with social scientists (@CeliaHeyes @paulgreenjunior @duncanjwatts @nfergus), activists and organizers (@MauriceWFP @cliff_notes) , . .
1
2
6
New preprint by @callfredaway and @mdhardy on the rational use of cognitive resources as a framework for modeling nudging, including designing optimal nudges!.
You know that feeling when you're trying to make a rational decision but all those pesky heuristics and biases make you do something silly? Wouldn't it be great if those biases helped us make ~better~ decisions instead?. (🧵).
0
0
4
. a poker champion (@AnnieDuke), an Olympic fencer, a kickboxing champion (@WonderboyMMA) and the co-founder of Instagram (@mikeyk). We hope you will enjoy listening to the series as much as we enjoyed making it!
0
0
2
This was a fun collaboration with @JQ_Zhu @ermgrant and @RTomMcCoy, featuring some of their recent work on Bayesian interpretations of neural networks (including LLMs) ..
0
2
2
@RubenLaukkonen The theory doesn't explain the accuracy effect. Perhaps computing accuracy involves a separate meta-cognitive process but it is very interesting how it could effect the feeling of being accurate after an Aha. An interesting puzzle to resolve in future work!.
1
0
1