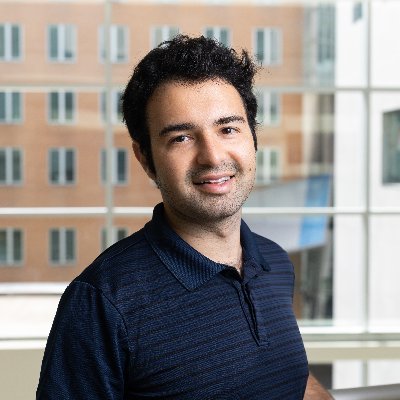
Aran Nayebi
@aran_nayebi
Followers
3K
Following
5K
Media
93
Statuses
1K
Asst Prof @CarnegieMellon Machine Learning (@mldcmu @SCSatCMU) | @BWFUND CASI Fellow | Building a Natural Science of Intelligence 🧠🤖 | Prev: @MIT, @Stanford
Pittsburgh, PA
Joined June 2010
I’m thrilled to be joining @CarnegieMellon’s Machine Learning Department (@mldcmu) as an Assistant Professor this Fall! . My lab will work at the intersection of neuroscience & AI to reverse-engineer animal intelligence and build the next generation of autonomous agents. Learn
76
44
931
Agree with Chalmers' take. As a neuroscience & AI researcher, I'm puzzled why this is remotely controversial though?. The "AI consciousness" claim rests on:.1. Brains are conscious. 2. Brain processes are physical. 3. Physical processes are Turing computable. (1) is.
this clip of me talking about AI consciousness seems to have gone wide. it's from a @worldscifest panel where @bgreene asked for "yes or no" opinions (not arguments!) on the issue. if i were to turn the opinion into an argument, it might go something like this:. (1) biology can.
148
53
373
1/8 Can we use embodied AI to gain insight into *why* neural systems are as they are?. In previous work👇, we demonstrated that a contrastive unsupervised objective substantially outperforms supervised object categorization at generating networks that predict mouse visual cortex.
Here we develop "Unsupervised Models of Mouse Visual Cortex".Co-lead with @NathanKong.w/ @ChengxuZhuang, Justin Gardner, @amnorcia, @dyamins. #tweetprint below 👇
4
62
354
1/ How do humans and animals form models of their world?. We find that Foundation Models for Embodied AI may provide a framework towards understanding our own “mental simulations”. 🧵👇. with awesome collaborators: @rishi_raj @mjaz_jazlab @GuangyuRobert
5
76
315
1/3 We release our ImageNet pretrained Recurrent CNN models, which currently best explain neural dynamics & temporally varying visual behaviors. Ready to be used with 1 line of code!.Models: Paper (to appear in Neural Computation):
Glad to share a preprint of our work on "Goal-Driven Recurrent Neural Network Models of the Ventral Visual Stream"!. w/ the "ConvRNN Crew" @jvrsgsty @recursus @KohitijKar @qbilius @SuryaGanguli @SussilloDavid @JamesJDiCarlo @dyamins . #tweetprint below👇
1
68
272
Glad to share a preprint of our work on "Goal-Driven Recurrent Neural Network Models of the Ventral Visual Stream"!. w/ the "ConvRNN Crew" @jvrsgsty @recursus @KohitijKar @qbilius @SuryaGanguli @SussilloDavid @JamesJDiCarlo @dyamins . #tweetprint below👇
Goal-Driven Recurrent Neural Network Models of the Ventral Visual Stream #biorxiv_neursci.
3
63
211
1/6 I usually don’t comment on these things, but @RylanSchaeffer et al.'s paper contains enough misconceptions that I thought it might be useful to address them. In short, effective dimensionality is not the whole story for model-brain linear regression, for several reasons:.
My 2nd to last #neuroscience paper will appear @unireps !!. 🧠🧠 Maximizing Neural Regression Scores May Not Identify Good Models of the Brain 🧠🧠. w/ @KhonaMikail @neurostrow @BrandoHablando @sanmikoyejo . Answering a puzzle 2 years in the making. 1/12.
3
30
162
Yesterday I successfully defended my PhD dissertation, "A Goal-Driven Approach to Systems Neuroscience". Especially grateful to @dyamins and @SuryaGanguli for advising me throughout this journey!. Defense Talk: Thesis:
15
10
150
1/ People study medial entorhinal cortex (MEC) because of its key role in navigation and memory. In our new paper we build neural network models that explain the full diversity of neural responses in MEC. To appear as a #NeurIPS2021 Spotlight! .Tweetprint below 👇🧠.
Explaining heterogeneity in medial entorhinal cortex with task-driven neural networks #biorxiv_neursci.
3
34
123
Here we develop "Unsupervised Models of Mouse Visual Cortex".Co-lead with @NathanKong.w/ @ChengxuZhuang, Justin Gardner, @amnorcia, @dyamins. #tweetprint below 👇
Unsupervised Models of Mouse Visual Cortex #biorxiv_neursci.
4
27
106
Excited to share our work "Two Routes to Scalable Credit Assignment without Weight Symmetry". Joint work with amazing collaborators Daniel Kunin, @jvrsgsty, @SuryaGanguli, @jbloom22, @dyamins.Paper: Code & weights: Tweetprint below:.
1
34
103
1/ Excited to share our new work on "Identifying Learning Rules From Neural Network Observables", to appear as a #NeurIPS2020 Spotlight!.w/ @sanjana__z @SuryaGanguli @dyamins. Paper: Code: Summary below 👇.
4
25
99
Thrilled to share this paper will be appearing in #NeurIPS2023 as a Spotlight!. Camera ready & models coming shortly. See you at @NeurIPSConf :).
1/ How do humans and animals form models of their world?. We find that Foundation Models for Embodied AI may provide a framework towards understanding our own “mental simulations”. 🧵👇. with awesome collaborators: @rishi_raj @mjaz_jazlab @GuangyuRobert
3
11
99
An overview of my past work & future directions is now available on the @mldcmu Youtube channel: It’s heavily based on my job talk, so it might be a useful resource for those on the faculty job market this year, as well as for prospective students!.
0
16
86
This is exactly the sort of understanding we want in neuroscience. Namely, a mathematically concise loss function that, when optimized, gives rise to the complexity/diversity of neural response profiles we see in a macroscopically (and functionally) significant brain area.
Pumped to share this work from my PhD, “A single computational objective drives specialization of streams in visual cortex”, just in time for the holidays! With the all star team @eshedmargalit , @cvnlab , @dyamins & @kalatwt .🔗:🧵: 1/n….
0
8
73
Indeed! This was one reason we released the ConvRNN model zoo: — because brain models must contend with dynamics (e.g., RNNs). PyTorch version coming very soon! While it likely won’t have pretrained ImageNet weights like the TF one above, it’ll make.
@GaryMarcus @tdietterich @MurthyLab @sapinker @Ozel_MN @DesplanLab A biggie is that the fly visual system is analogous to a *recurrent* convolutional net, which is not so popular in AI. It's recurrent through and through, starting with the photoreceptors.
1
6
66
Really honored to be selected as a recipient of this year’s CASI award, which will help fund my incoming lab @mldcmu for the next 5 years!. Read more here about our plans at the intersection of neuroscience & AI:
Announcing the 2024 Career Award at the Scientific Interface recipients #bwfcasi.
5
4
64
Temporarily escaped the winter storm in Boston to give a talk on "Goal-Driven Models of Physical Understanding" at @Stanford's NeuroAI course (CS375). Slides here: Always good to be back in my intellectual home! ☀️
1
4
58
1/ Interested in how task-driven neural networks help us understand the diverse cell types in medial entorhinal cortex (MEC), a brain area that plays a key role in navigation & memory? . Stop by #NeurIPS2021 poster session 6 tomorrow (8:30 am PT, Spot F0)!.
1
12
53
Excited to share our work on Mouse Visual Cortex is now published in @ploscompbiol: #ploscompbiolauthor. We also release our SOTA pretrained models & our Pytorch library of SSL methods so others can build their own models easily:
1/8 Can we use embodied AI to gain insight into *why* neural systems are as they are?. In previous work👇, we demonstrated that a contrastive unsupervised objective substantially outperforms supervised object categorization at generating networks that predict mouse visual cortex.
1
12
51
My talk on this work at Oxford, as part of the CCN '23 GAC, is now available: The main point is that by comparing to neural data from frontal cortices where the animal performs an active cognitive task, we are quickly lead into needing new modeling ideas.
1/ How do humans and animals form models of their world?. We find that Foundation Models for Embodied AI may provide a framework towards understanding our own “mental simulations”. 🧵👇. with awesome collaborators: @rishi_raj @mjaz_jazlab @GuangyuRobert
2
8
49
1/4 My talk @MIT_CBMM is available now, where I discuss how self-supervised learning of visual representations that are reusable for downstream Embodied AI tasks may be a unifying organizing principle to understand neural coding across multiple species and brain areas. 🧵👇.
[video] "Using Embodied AI to help answer 'why' questions in systems neuroscience". @aran_nayebi Aran Nayebi, MIT.
1
8
49
If you're attending #NeurIPS2023 this year, stop by Poster #417 this Thursday Dec. 14 from 8:45-10:45 am, where I'll be presenting this work!.@NeurIPSConf
1/ How do humans and animals form models of their world?. We find that Foundation Models for Embodied AI may provide a framework towards understanding our own “mental simulations”. 🧵👇. with awesome collaborators: @rishi_raj @mjaz_jazlab @GuangyuRobert
1
2
48
Excited to share an 8-min overview of our lab's planned work at the intersection of neuroscience & AI, building embodied agents to reverse-engineer natural intelligence, as part of @SCSatCMU's new faculty lightning talks!.
1
5
47
I will be presenting this work at #CCN2023 this Thursday at Poster P-1B.114 from 17:00-19:00 (, as well as on Friday 08:35 at the GAC on "Comparing artificial and biological networks" (. Stop by if interested! @CogCompNeuro.
1/ How do humans and animals form models of their world?. We find that Foundation Models for Embodied AI may provide a framework towards understanding our own “mental simulations”. 🧵👇. with awesome collaborators: @rishi_raj @mjaz_jazlab @GuangyuRobert
1
8
48
In this @StanfordAILab blog post👇I discuss our recent work ( on a model-based approach towards identifying the brain’s learning algorithms. If interested, stop by our #NeurIPS2020 poster: Session 6, Thursday 9-11am PST! (.
A major long-term goal of computational neuroscience is to identify the brain's learning algorithms. Can we use artificial neural networks to guide this discovery?. Check out this blog post by @aran_nayebi about recent work accepted at #NeurIPS2020:
0
11
45
Unfortunately, @RylanSchaeffer 's responses seem to obscure key points rather than clarify them. I’d like to offer some clarification here. Your claim that the field has "devolved into overfitting," along with the implication that highly linearly predictive models are able to.
I wanted to wait before responding to @aran_nayebi 's post ( because it seemed like tempers were high, and to steal a phrase I learned this week from a friend:. The goal is to create light 💡 not heat 🔥. - To briefly restate our overarching perspective,.
2
2
43
1/15 This is an important point worth underscoring. As I’ll elaborate here below -- there is actually a lot of shared perspective between critiques of NeuroAI with the main considerations of those who practice it. It also leads to some new directions that I'll note!🧵👇.
@jmourabarbosa @martin_schrimpf @TimKietzmann @JamesJDiCarlo @dyamins @NKriegeskorte @neurograce I'm afraid I must bust out my go-to reaction. 😉. The utility of the 3 papers here, as with other similar ones, is to show what our current models are not correctly capturing. But, they do not demonstrate that multi-layer ANNs are the wrong tool for modelling the brain.
1
10
39
Great to see our work on developing methods towards identifying the brain’s learning rules featured in @TheEconomist today! . Thanks @AinslieJstone for writing this piece!. For more details, here's the original tweetprint 🧵👇:
Designing experiments to determine which algorithm is at play in the brain is surprisingly tricky. For some researchers at Stanford University, this seemed like a problem AI could solve 🧠.
1
12
39
This was one of the first books that got me into neuroscience! Saw it in the Stanford bookstore my senior year. Remains a classic.
To celebrate the 10th anniversary of our book we are planning a brief essay on what has followed it. Please comment: how well do new facts conform to the principles it enunciates? Have you found violations? Send us your new examples, doubts, and any new principles.
1
2
36
Nice to see our latest work on mental simulation featured on the front page of @MIT News today!. If interested in more detail, my recent @MIT_CBMM talk explains some of the motivations of using Self-Supervised Learning for Embodied AI to study the brain:
Two papers by researchers at the K. Lisa Yang ICoN Center have found that “self-supervised” models, which learn about their environment from unlabeled data, can show activity patterns similar to those of the mammalian brain. .@ScienceMIT @mitbrainandcog.
1
2
36
Got to meet @stephen_wolfram today and talk to him about LLMs, neural architecture search, and NeuroAI more broadly!. Thank you @neuranna @eghbal_hosseini and @maria_ryskina for organizing!
1
0
35
I find it sobering that hardly any neuroscience was useful or needed to build this. The science of intelligence will come about by studying models that *actually work*, not toy systems that can't perform any realistic behaviors.
This is so surreal. We've replaced horses with robots. Adam Savage from Mythbusters built a rickshaw pulled by Boston Dynamics robot, Spot (the one that can now speak using ChatGPT).
2
1
33
@ylecun @Meta FWIW, we find strong evidence against pixel-level generative models in the brain's "physics engine". Latent future prediction matches brain data best, but not all latents are equal. The best latents are relevant to embodiment, trained via SSL on Ego4D:.
1/ How do humans and animals form models of their world?. We find that Foundation Models for Embodied AI may provide a framework towards understanding our own “mental simulations”. 🧵👇. with awesome collaborators: @rishi_raj @mjaz_jazlab @GuangyuRobert
0
3
35
"Can robotics help us understand the brain?". A fun short video on our recent #NeurIPS2023 Spotlight paper! . Full paper: Tweetprint:
#MITTeachMeSomething.Can robotics help us understand the brain?. @aran_nayebi Aran Nayebi, ICoN Postdoctoral Fellow, MIT Dept. of Brain and Cognitive Sciences Want to learn more?. #MIT #TeachMeSomething #robotics #neuroscience #AI #brain #understanding
1
1
30
Great to finally see this out -- 5 years in the making!.
1/Our paper @NeuroCellPress "Interpreting the retinal code for natural scenes" develops explainable AI (#XAI) to derive a SOTA deep network model of the retina and *understand* how this net captures natural scenes plus 8 seminal experiments over >2 decades
1
0
28
Looking forward to speaking about model improvements from reverse-engineering natural intelligence at @GoogleAI tomorrow!.
4
0
30
I guess this officially means we're physicists now 😂.(credit to the @mldcmu grad students for putting this up!)
1
2
28
If you're attending #cosyne2023, stop by our Poster III-002 👇 this Saturday at 8:30 pm on "Mouse visual cortex as a limited resource system that self-learns an ecologically-general representation"!. w/ @NathanKong @ChengxuZhuang Justin Gardner @amnorcia @dyamins
2
7
27
Looking forward to speaking at the #SfN19 Minisymposium "Artificial Intelligence and Neuroscience"! I'll be presenting our work on task-driven recurrent convolutional models of higher visual cortex dynamics. Monday Oct. 21, 9:55-10:15 am, Session 261, Room S406A.
1
3
27
@GalacticArcanum I hope the gods guarding the microtubules in the 11th dimension don't smite me!.
3
0
26
Saw this on the way to giving a talk to the incoming @CMU_Robotics students this morning. It’s not everyday you see your intellectual heroes encapsulated in one photo! 🤩
0
0
26
Excited to visit & share my work with the @pennbioeng community next week!.
There is no #PennBioengineering seminar this week. Next week, @aran_nayebi of @MIT will speak on systems neuroscience. Please note that this seminar will be held in Wu & Chen Auditorium (Levine 101) instead of our normal location. Bio & abstract:
0
2
26
Really enjoyed speaking with @alisonmsnyder about the importance of studying embodied cognition in both neuroscience & AI!.
0
3
26
The core points are: .1. mental simulation is relevant to a future latent space, but.2. this latent is highly constrained -- it doesn't appear to consist of bespoke object slots or pay attention to fine-grained details (e.g. pixelwise), but has to be reusable on *dynamic* scenes.
1/ How do humans and animals form models of their world?. We find that Foundation Models for Embodied AI may provide a framework towards understanding our own “mental simulations”. 🧵👇. with awesome collaborators: @rishi_raj @mjaz_jazlab @GuangyuRobert
0
6
27
Video from my #cosyne19 talk is out now, on measuring and modeling the dynamics of hundreds of synapses in-vivo. Joint work with fantastic collaborators @melandrocyte (who heroically collected this data), @SuryaGanguli, Tianyi Mao, and @HainingZhong.
0
4
23
What types of neuroscience data should we collect in order to identify the learning rule operative within a neural circuit? . Stop by #cosyne21 poster 1-116 Wednesday, 1B (12-2 pm PT) and 1C (4-6 pm PT), where I’ll be discussing our model-based approach to this question.
1/ Excited to share our new work on "Identifying Learning Rules From Neural Network Observables", to appear as a #NeurIPS2020 Spotlight!.w/ @sanjana__z @SuryaGanguli @dyamins. Paper: Code: Summary below 👇.
1
3
25
Aside #2: Whether the Turing machine (TM) for neural processes in (3) is classical or quantum affects the feasibility of digital brain copies. If a quantum TM is needed, we'd need the quantum state of every molecule, making accurate digital copies impossible due to the No-Cloning.
3
0
24
#NeurIPS2023 Camera Ready out now: Pretrained model weights & code:
1/ How do humans and animals form models of their world?. We find that Foundation Models for Embodied AI may provide a framework towards understanding our own “mental simulations”. 🧵👇. with awesome collaborators: @rishi_raj @mjaz_jazlab @GuangyuRobert
0
7
24
5/ We found @rishi_raj’s dense neurophysiological data to be an especially strong differentiator of models. In fact, only *one* model class generalizes & predicts these data well – those trained to future predict in the latent space of foundation models optimized for *videos*!
1
2
23
Really glad to see this out! In this work co-lead with Josh Melander, we measure & analyze the dynamics of hundreds of synaptic weights in-vivo over the course of approximately a month.
Our new preprint is out. It fills a gap in the field. Great collaboration with Tianyi Mao and @SuryaGanguli lab. Congrats to the team: Josh, @aran_nayebi, Bart, Dale, and Maozhen.
1
4
20
8/10 As time went on, I forgot about the manuscript, and my interests shifted to neuroscience. I only realized I still had it in my files this weekend, after an article by @stephen_wolfram triggered my memory:
1
1
21
I remember Jay telling me in grad school how the 1986 Rumelhart et al. backprop paper came about—not from trying to guess "biological" learning rules, but from a push to directly optimize for tasks, which proved way more effective. Glad to see it online:
@SebastianSeung Backprop is moreso an efficient proxy for "evolution", to evolve an ANN's weights towards a particular goal (within our lifetimes 😅). It's likely overkill for use in an organism's lifetime learning algo, esp. considering the strong inductive biases in the brain's circuitry.
3
1
20
7/10 It was also interesting to see the various recollections of Turing and the early days of computing as I typed up Gandy's manuscript. We all agreed it would be valuable to put the typeset version online at some point, but decided to hold off until the @AlanTuringYear finished.
1
0
20
5/10 Sol graciously contacted logicians he knew requesting if anyone had a copy on hand, and we finally managed to get ahold of it from the late @SBarryCooper, and a former Ph.D. student of Gandy's, Philip Welch.
1
0
18
After some initial hurdles, I have now put Gandy's previously unpublished manuscript on arXiv! I hope that this will further aid in its historical preservation.
1/10 Ten years ago, I received a handwritten manuscript by Alan Turing’s only PhD student, Robin Gandy. He wrote it a couple years before passing away in 1995. AFAIK, it’s never before been in print, so I typeset my copy & put it online here: 🧵👇.
0
0
16
Stumbled on a 2011 talk by @AndrewYNg at Stanford where he advocates for using the brain to inspire AI solutions. He presents early results showing how trained neural nets qualitatively develop biological receptive fields—a nice anticipation of NeuroAI!.
0
2
19
@SuryaGanguli @stanislavfort @KordingLab @tyrell_turing @hisspikeness @stanislavfort The best we could get in our ICML '20 paper ( was to match backprop performance on ImageNet up to 50 layers using a purely layer-local learning rule (Info. Alignment, cf. Figure 3). If you allow non-locality (but still no strict weight
1
0
17
In this note, we critically analyze recent claims made about the fragility of grid cell emergence in trained neural networks, and show they robustly appear when prior theory predicted they should. More details below👇.
1/ Our new preprint on when grid cells appear in trained path integrators w/ Sorscher @meldefon @aran_nayebi @lisa_giocomo @dyamins critically assesses claims made in a #NeurIPS2022 paper described below. Several corrections in our thread ->.
0
5
18
I'll be giving a talk @SfNtweets this Wednesday 1:45 pm on our Mental Simulation work (TL;DR below👇), at the Computational Models nanosymposium. Full schedule here:
0
5
18
Yep — across species, the hard problem that brains solve exactly fall in the domain of robotics — enabling meaningful actions that ensure the animal’s survival in the physical world. The toy tasks typically used in comp. neuro. fall far short of this mark (along with modern DL).
I have come to believe that building some kind of functioning robot should be a compulsory educational requirement for every neuroscientist.
1
1
17
I’m blessed to have had such supportive mentors and colleagues who helped me make this dream come true. I’m especially grateful to @dyamins & @SuryaGanguli for their guidance and support during my PhD, and to @GuangyuRobert & @mjaz_jazlab during my postdoc, as well as many.
1
0
17
Attending @icmlconf and interested in scaling up biologically plausible learning rules? Stop by our virtual #ICML2020 poster Tuesday, July 14, 0700-0745 PT or 2000-2045 PT for a Q&A!. Further details (and video co-presented with @jvrsgsty) here:
Excited to share our work "Two Routes to Scalable Credit Assignment without Weight Symmetry". Joint work with amazing collaborators Daniel Kunin, @jvrsgsty, @SuryaGanguli, @jbloom22, @dyamins.Paper: Code & weights: Tweetprint below:.
0
3
16
Excited to be on the panel for this tomorrow! Stop by if you’re at #ICLR2024!.
Really looking forward to tomorrow's First Workshop on Representational Alignment (Re-Align) at #ICLR2024! . Check out the schedule at w/ @ermgrant @sucholutsky @lukas_mut @achterbrain.
0
0
15
2/6 The spectral theory (pictured below) quite literally includes an alignment term of the model PCs being aligned with the neural data. @kendmil & @ShahabBakht also nicely point this out:
@ShahabBakht @RylanSchaeffer @canatar_a Agree -- in the formula quoted above from Canatar et al 2023, alignment of the model PC's with the neural data plays a critical role, it is not just the model variances which determine dimensionality; in fact, Figs SI5.10-SI5.12 of that paper show dim doesn't predict error. ??.
1
1
15
@stanislavfort @SuryaGanguli @KordingLab @tyrell_turing @hisspikeness It's certainly a useful step to have these alternatives that scale with the current (rate-based) architectures. It subsequently led us to ask what we would need to measure in the actual brain to separate candidate learning rule hypotheses (NeurIPS '20): I.
2
3
14
Also, the "solutions" you propose are exactly what the field of NeuroAI has been doing since its inception:.
We've been thinking hard about what positive, constructive paths forward look like and we have some initial ideas:. (1) Clearly identifying what similarity to a specific neural system means. (2) Choosing bespoke metrics for the neural system of interest rather than a fixed.
0
0
14
💯💯 captures exactly how I feel this week.
It's always painful to see a community dedicate time & energy trying to make sense of an overhyped sloppy result. Just a huge waste of time in most cases, where one side acts in good faith while the other spews quite a bit of nonsense (and gets rewarded with praise from those.
3
0
13
@AndrewLampinen Yep, that asterisk is crucial! We wouldn't expect this with MLPs, for example. Innovating with new architectures that achieve the successes of CNNs & Transformers is challenging in general.
2
0
14
I can’t wait to join the fantastic @mldcmu and @cmuneurosci communities, an ideal place for NeuroAI to thrive. Stay tuned!.
1
0
14
I'll be presenting this work as a lightning talk at #ICDL2024 in UT Austin tomorrow at 3:15 pm CT! Stop by and say hi if you're attending!. Full schedule here:
1/ How do humans and animals form models of their world?. We find that Foundation Models for Embodied AI may provide a framework towards understanding our own “mental simulations”. 🧵👇. with awesome collaborators: @rishi_raj @mjaz_jazlab @GuangyuRobert
0
0
14
The limitations of human language are also applicable to systems neuroscience, since many neural response profiles exhibit difficult to interpret functional roles beyond single experimental variables. This is why task-optimization is a useful formal framework to engage w/ this.
Light and matter are both single entities, and the apparent duality arises in the limitations of our language. It is not surprising that our language should be incapable of describing the processes occurring within the atoms, for, as has been remarked, it was invented to describe
0
1
13